Refining Seismic Data Processing Techniques for Precision and Efficiency
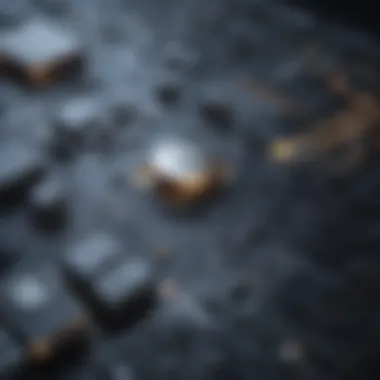
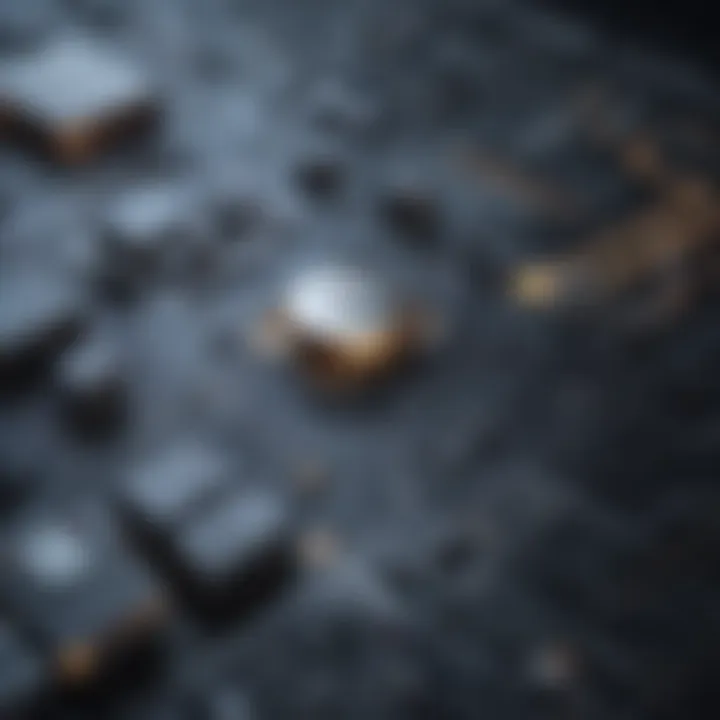
Overview of Seismic Data Processing
Seismic data processing stands at the forefront of modern technological advancements, revolutionizing the way subsurface structures are imaged and interpreted. The intricate methods and techniques employed in this field are pivotal in enhancing the precision and efficiency of data interpretation, ultimately leading to significant developments in various industries. Understanding the evolution and historical background of seismic data processing provides insightful context into the sophisticated procedures utilized today.
Fundamentals of Seismic Data Processing
Delving into the fundamentals of seismic data processing unveils a realm of core principles and theories that underpin this intricate domain. Exploring key terminologies and definitions is essential to grasp the essence of seismic data interpretation fully. Building a solid foundation of basic concepts is crucial for individuals aiming to navigate through the complexities of processing seismic data with acumen and expertise.
Practical Applications in Seismic Data Processing
Real-world case studies and applications serve as guiding beacons in the vast sea of seismic data processing. Through demonstrations and hands-on projects, individuals can witness firsthand the pragmatic implications of refined processing techniques. Incorporating code snippets and implementation guidelines further amplifies the practicality of applying seismic data processing in various scenarios, enriching one's skill set and proficiency.
Advanced Innovations and Emerging Trends
Embracing advanced topics and the latest trends in seismic data processing propels individuals towards the forefront of cutting-edge developments in the field. The exploration of advanced techniques and methodologies sheds light on innovative approaches that redefine traditional paradigms. By delving into future prospects and upcoming trends, individuals can stay primed for the dynamic shifts and revolutions in seismic data processing, securing a competitive edge in the industry.
Tips and Resources for Enthusiastic Learners
For those keen on delving deeper into the realms of seismic data processing, a plethora of resources await to nurture their curiosity and expertise. Recommended books, courses, and online resources act as pillars of knowledge, providing comprehensive insights and practical guidance. Further enriching one's learning experience, tools and software tailored for practical usage equip individuals with the necessary arsenal to embark on a journey of continuous learning and growth.
Introduction
In this section, we delve into the foundational elements that underpin the intricate process of seismic data processing. Understanding seismic data processing is vital as it forms the backbone of generating accurate subsurface images crucial for various applications. Through a systematic approach from acquisition to interpretation, every phase acts as a linchpin in ensuring the precision and efficiency of seismic data interpretation.
Understanding Seismic Data Processing
Importance of Seismic Data
Shedding light on the significance of seismic data, it is pivotal due to its role in deciphering the subsurface composition with accuracy. The importance lies in the ability to unveil hidden geological structures and potential resources beneath the surface. This facet of seismic data is indispensable in making informed decisions regarding resource exploration and extraction. Despite its complexity, seismic data offers a comprehensive understanding of subsurface dynamics, making it a crucial aspect of this article. Its distinctive feature lies in its ability to provide detailed insights into the geological composition but may pose challenges in interpretation due to its intricate nature.
Goals of Seismic Data Processing
The goals of seismic data processing revolve around refining techniques to enhance the precision and efficiency of interpreting subsurface images. These goals aim to streamline the process, reduce ambiguity in interpretation, and minimize errors in data analysis. By setting clear objectives in seismic data processing, professionals can achieve a higher level of accuracy in understanding subsurface structures. The bespoke nature of these goals makes them a quintessential choice for this article, as they drive the narrative towards achieving optimal results. The unique feature of these goals lies in their ability to revolutionize the interpretation process but may present challenges in implementation due to the intricate nature of subsurface data.
Challenges and Opportunities
Complexity of Subsurface Structures
The complexity of subsurface structures presents a fascinating yet challenging realm in seismic data processing. Understanding the intricate layers and formations beneath the surface is essential for accurate interpretation. This complexity adds richness to the data by capturing detailed geological features that might otherwise go unnoticed. However, delving into these intricate structures demands a high level of expertise and sophisticated algorithms to decipher the data accurately. The advantage of this complexity is the depth of information it provides but may pose challenges in data processing due to its sheer intricacy.
Technological Advancements
Technological advancements play a pivotal role in shaping the landscape of seismic data processing. These innovations have opened up new possibilities for more precise imaging and interpretation of subsurface structures. By leveraging cutting-edge technologies, professionals can overcome traditional limitations and delve deeper into the intricacies of seismic data. The advantage of technological advancements lies in their ability to enhance data processing speed and accuracy, leading to more reliable results. However, adopting these technologies may come with challenges such as high implementation costs and the need for specialized training in handling advanced equipment.
Data Acquisition
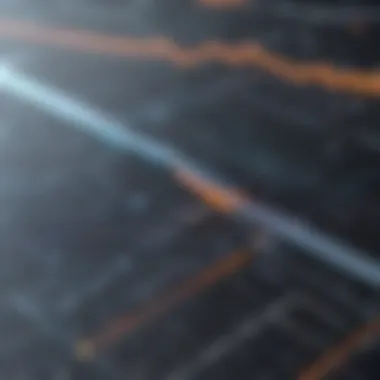
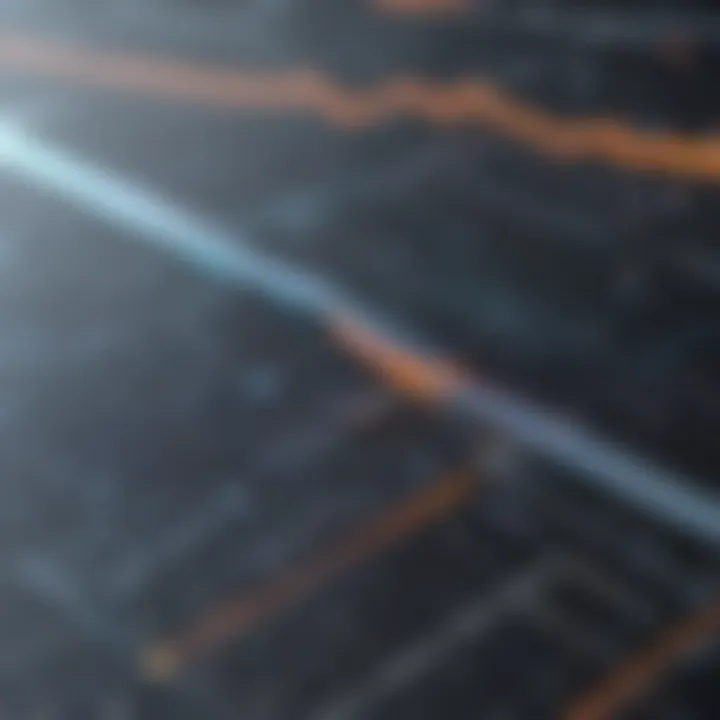
In the realm of seismic data processing for precision and efficiency, the pivotal stage of Data Acquisition warrants meticulous attention. This phase serves as the foundation upon which the entire process unfolds, emphasizing the criticality of acquiring high-quality data. Acquiring precise data sets is imperative for generating accurate subsurface images, a fundamental requirement for a myriad of applications ranging from oil exploration to geological studies. The accuracy and reliability of the acquired data directly impact the efficacy of subsequent processing stages, making Data Acquisition a linchpin in the seismic data processing workflow.
Seismic Sensor Technology
Introduction to Seismic Sensors
Delving into the intricacies of Introduction to Seismic Sensors unveils a key aspect of seismic data processing. The utilization of advanced sensor technology plays a pivotal role in enhancing the efficiency and accuracy of data collection. The distinctive characteristic of Introduction to Seismic Sensors lies in its ability to capture seismic waves with precision, providing detailed insights into subsurface structures. This aspect is instrumental in obtaining high-resolution data essential for in-depth analysis and interpretation. Despite its numerous advantages, Introduction to Seismic Sensors may present challenges such as sensitivity to environmental conditions, requiring robust calibration procedures to optimize performance.
Types of Seismic Sensors
Exploring the nuances of Types of Seismic Sensors sheds light on the diverse array of sensor technologies available in the seismic data processing domain. Each type offers unique capabilities tailored to specific operational requirements, contributing significantly to the overarching goals of precision and efficiency. The distinguishing feature of Types of Seismic Sensors lies in their adaptability to varying terrains and depth ranges, enabling comprehensive data collection across different geological settings. While the versatility of Types of Seismic Sensors enhances data acquisition capabilities, it also necessitates a thorough understanding of operational considerations to mitigate potential limitations and optimize performance.
Field Operations
Survey Planning
Survey Planning emerges as a strategic element in the seamless execution of field operations within the realm of seismic data processing. The meticulous planning of survey parameters and layout is crucial for ensuring comprehensive data coverage while minimizing operational complexities. Survey Planning - an indispensable component in data acquisition - dictates the success of subsequent processing stages through its influence on data quality and spatial resolution. The key attribute of Survey Planning lies in its ability to optimize resources and streamline data collection processes, paving the way for efficient analysis and interpretation. However, challenges such as logistical constraints and terrain variability necessitate prudent planning and adaptive strategies to overcome operational hurdles and maximize data acquisition efficiency.
Data Collection Techniques
Data Collection Techniques play a significant role in facilitating the acquisition of high-fidelity seismic data essential for precise imaging and interpretation. The methodological integration of cutting-edge techniques ensures the comprehensive capture of subsurface data while minimizing noise and artifacts. The hallmark feature of Data Collection Techniques is their ability to adapt to diverse environmental conditions and seismic objectives, enhancing the efficacy of data acquisition processes. Despite their advantages, these techniques require meticulous calibration and monitoring to maintain data integrity and validity throughout the collection phase, underscoring the importance of standardized protocols and quality assurance measures.
Preprocessing Stage
In this article, the Preprocessing Stage holds a paramount importance as it acts as the foundation for subsequent data processing steps. Preprocessing aims to clean and refine raw seismic data, preparing it for advanced analysis. By meticulously addressing noise, inconsistencies, and unwanted artifacts, the Preprocessing Stage significantly enhances data quality, leading to more accurate interpretation results. Efficient preprocessing streamlines the entire seismic data processing workflow, saving time and resources while boosting the overall efficiency of the seismic exploration process.
Noise Removal
Filtering Techniques
Filtering Techniques play a crucial role in the Noise Removal process within seismic data processing. These techniques involve applying various filters to eliminate unwanted noise signals, thereby enhancing the clarity and reliability of seismic data. One key characteristic of Filtering Techniques is their ability to target specific noise frequencies, allowing for precise noise reduction without altering essential seismic information. These techniques are highly beneficial in this article as they help in improving data accuracy and interpretation quality. The unique feature of Filtering Techniques lies in their adaptability to different types of noise sources, offering flexibility in addressing varying noise complexities in seismic data processing.
Signal Enhancement
Signal Enhancement serves as another vital aspect of Noise Removal, focusing on amplifying desired signals while suppressing noise interference. The key characteristic of Signal Enhancement lies in its ability to highlight subtle seismic features that might be masked by noise, improving the overall data quality and interpretive insights. In the context of this article, Signal Enhancement proves to be a valuable choice in optimizing signal-to-noise ratios and extracting valuable information from seismic data. The unique feature of Signal Enhancement is its capability to selectively enhance specific signal attributes, enabling a more detailed analysis and interpretation of subsurface structures.
Data Quality Control
The aspect of Data Quality Control is essential in ensuring the reliability and accuracy of seismic data used for interpretation and decision-making processes. Quality Assessment involves evaluating various data metrics to assess the overall quality and fidelity of seismic data. This step is crucial in identifying potential anomalies or inconsistencies that could impact the interpretation outcome. In this article, Quality Assessment stands out as a fundamental choice due to its role in validating the authenticity of processed seismic data and certifying its suitability for precise interpretation purposes.
Error Correction Methods
Error Correction Methods contribute significantly to refining seismic data quality by addressing errors and discrepancies that may arise during processing. These methods focus on rectifying inaccuracies, misalignments, or inconsistencies in the data to align with geological realities accurately. The key characteristic of Error Correction Methods is their ability to enhance the reliability and trustworthiness of seismic interpretations by rectifying potential inaccuracies. In this article, leveraging Error Correction Methods proves advantageous in mitigating uncertainties and ensuring the precision and accuracy of subsurface imaging results.
Processing Techniques
In the realm of seismic data processing, the utilization of processing techniques holds paramount significance. These techniques serve as the bedrock for refining raw seismic data into interpretable subsurface images that are crucial for diverse applications. By employing sophisticated algorithms and methodologies, seismic data analysts can enhance the precision and efficiency of data interpretation, leading to more accurate geological insights and informed decision-making processes. The key elements of processing techniques revolve around mitigating noise, improving signal quality, and spatially organizing seismic data for comprehensive analysis.
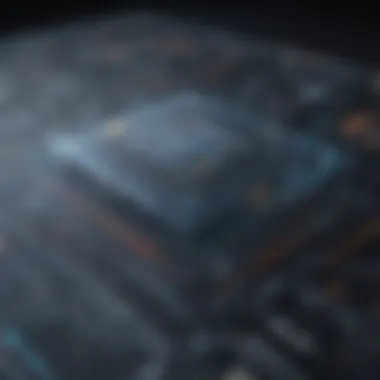
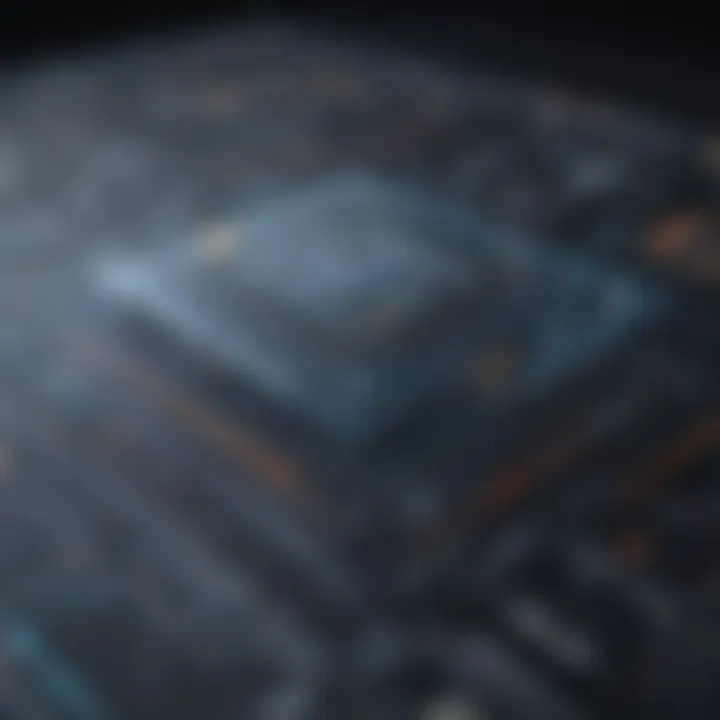
Migration Algorithms
Kirchhoff Migration
Kirchhoff Migration stands out as a pivotal component within the processing techniques domain, contributing significantly to the overarching goal of enhancing seismic data processing. The standout characteristic of Kirchhoff Migration lies in its adeptness at accurately imaging complex subsurface structures with high resolution. Due to its versatile nature and adaptability to various geological settings, Kirchhoff Migration emerges as a preferred choice for seismic data interpretation in this article. Its unique feature lies in its ability to handle intricate subsurface features effectively, although it may entail computational demands and challenges in handling steeply dipping events.
Reverse Time Migration
Reverse Time Migration represents another vital aspect of processing techniques, playing a crucial role in achieving the desired precision and efficiency in seismic data processing. The key characteristic of Reverse Time Migration lies in its capability to handle complex wave propagation patterns and provide detailed images of subsurface structures. This method is favored for its ability to handle steeply dipping structures and salt bodies effectively, making it a popular choice for enhancing seismic data interpretation in this article. However, Reverse Time Migration may come with computational complexities and high resource requirements, impacting its efficiency in certain scenarios.
Velocity Analysis
Dix Conversion
Dix Conversion emerges as a fundamental segment of velocity analysis, enriching the overall seismic data processing with its unique contributions. The key characteristic of Dix Conversion lies in its ability to transform seismic travel times into quantitative information about subsurface velocities, enabling more accurate imaging of geological formations. Dix Conversion is a beneficial choice for this article due to its essential role in calibrating seismic data and optimizing subsurface imaging. However, it may pose challenges in handling variations in seismic velocities and complex geological settings.
Tomographic Velocity Analysis
Tomographic Velocity Analysis plays a substantial role in enhancing seismic data processing by providing in-depth insights into subsurface velocity variations. The key characteristic of this analysis technique is its ability to create detailed velocity models, aiding in the accurate interpretation of seismic data. Tomographic Velocity Analysis is preferred in this article for its efficiency in characterizing complex subsurface structures and improving imaging accuracy. Nevertheless, challenges may arise in handling large datasets and complex geological features, impacting the overall processing efficiency.
Interpretation Phase
In the realm of seismic data processing, the Interpretation Phase stands as a critical juncture that bridges data processing with actionable insights. This phase is where the processed seismic data is meticulously analyzed to decipher the subsurface structures accurately. It plays a pivotal role in determining the presence of potential reservoirs, faults, and other geological features essential for decision-making in various industries. By focusing on Structural Interpretation and Attribute Analysis, the Interpretation Phase aims to extract valuable insights that drive precision and efficiency in subsurface imaging processes.
Structural Interpretation
Fault Detection
Fault Detection within Structural Interpretation emerges as a fundamental element in the analysis of seismic data. This technique specifically targets the identification of fault lines and fractures in the subsurface layers with precision. Fault Detection aids in understanding the structural complexities of the Earth's crust, which is crucial for reservoir characterization and seismic hazard assessment. Its ability to pinpoint fault lines accurately enhances the predictive nature of seismic data interpretation. One key characteristic of Fault Detection is its capacity to distinguish subtle shifts in geological formations, allowing for a detailed representation of subsurface structures. The uniqueness of Fault Detection lies in its exceptional accuracy in identifying fault networks, providing a solid foundation for robust geological interpretations. While advantageous in revealing fault geometries, this technique may present challenges in differentiating between fault-related seismic attributes and noise in complex subsurface environments.
Horizon Tracking
Within Structural Interpretation, Horizon Tracking plays a significant role in delineating stratigraphic boundaries in seismic data. This method involves tracing specific seismic reflections across different profiles, enabling the interpretation of geological layering and depositional sequences. The key characteristic of Horizon Tracking lies in its capacity to establish the continuity of geological horizons, aiding in subsurface mapping and reservoir characterization. This technique is a popular choice in seismic interpretation due to its ability to visualize complex geological structures accurately. The uniqueness of Horizon Tracking lies in its ability to track discontinuities in seismic data, allowing for the identification of stratigraphic features with precision. While advantageous in improving stratigraphic interpretations, Horizon Tracking may face limitations in scenarios with obscured reflectors or overlapping seismic signals.
Attribute Analysis
Amplitude Variations
Amplitude Variations are a crucial aspect of Attribute Analysis, contributing significantly to enhancing the understanding of subsurface properties within seismic data. This analysis focuses on variations in signal amplitudes across seismic traces, providing insights into structural heterogeneities and fluid content within geological formations. The key characteristic of Amplitude Variations is its sensitivity to changes in rock properties, allowing for the differentiation of lithologies and fluid reservoirs. This technique's uniqueness lies in its ability to detect subtle amplitude changes, aiding in reservoir characterization and hydrocarbon exploration. While advantageous in highlighting reservoir potentials, Amplitude Variations may encounter challenges in differentiating between amplitude anomalies caused by lithology variations and acquisition-related noise.
Frequency Content Analysis
Frequency Content Analysis serves as a vital component of Attribute Analysis, offering valuable information on the frequency distribution of seismic data. By analyzing the spectral content of seismic signals, this technique facilitates the detection of structural features and lithological properties in subsurface formations. The key characteristic of Frequency Content Analysis is its ability to identify seismic facies variations based on frequency patterns, aiding in reservoir delineation and lithology prediction. This technique's uniqueness lies in its capacity to reveal subsurface heterogeneities through frequency-related attributes, enhancing reservoir characterization and geological modeling. While advantageous in defining reservoir architecture, Frequency Content Analysis may encounter limitations in environments with complex frequency spectra or overlapping seismic responses.
Advanced Techniques
In the realm of seismic data processing, the utilization of advanced techniques is paramount to ensuring precision and efficiency in subsurface imaging. These sophisticated methods play a pivotal role in refining seismic data interpretation processes, leading to more accurate results with enhanced clarity and resolution. By employing advanced techniques, seismic data analysts can overcome challenges related to complex geological structures and optimize the overall data processing workflow. The integration of cutting-edge technologies and innovative approaches enables the extraction of valuable insights from seismic data, facilitating informed decision-making in various applications ranging from oil and gas exploration to environmental monitoring and geotechnical studies.
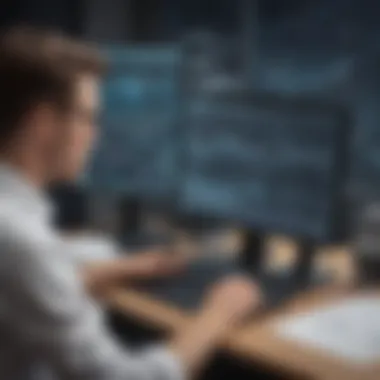
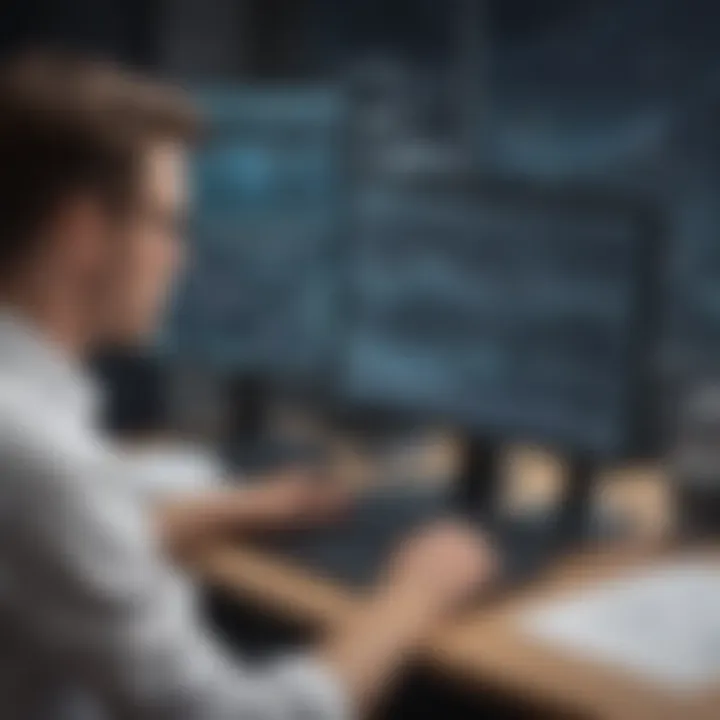
Machine Learning Applications
Supervised Learning Models
When delving into the realm of supervised learning models within the context of seismic data processing, one cannot overlook their indispensable contribution to enhancing interpretation accuracy and efficiency. Supervised learning models excel in training algorithms based on labeled datasets, allowing for the prediction of outcomes when new data is presented. The key characteristic of supervised learning models lies in their ability to learn patterns and relationships from labeled examples, which proves highly beneficial in classification and regression tasks within seismic data analysis. Leveraging supervised learning models in this article significantly streamlines the data interpretation process, offering a structured approach that enhances decision-making and interpretation quality. Despite their efficacy, supervised learning models may face challenges with overfitting or the need for extensive labeled data, which should be carefully considered in the context of seismic data processing optimization.
Unsupervised Techniques
In the domain of unsupervised techniques for seismic data analysis, their notable contribution towards uncovering hidden patterns and structures within unlabeled datasets is crucial for optimizing interpretations effectively. Unsupervised techniques stand out for their ability to identify inherent structures and relationships within complex seismic datasets without the need for labeled information, offering valuable insights into data organization and segmentation. The key characteristic of unsupervised techniques lies in their exploratory nature, facilitating data clustering, anomaly detection, and pattern recognition in seismic data without prior guidance. Embracing unsupervised techniques in this article enhances the holistic view of seismic data, promoting a deeper understanding of subsurface characteristics and anomalies that might go unnoticed with traditional methods. However, challenges such as interpretation complexity and algorithm sensitivity need attention when integrating unsupervised techniques into seismic data processing workflows.
Deep Learning in Seismic Interpretation
Convolutional Neural Networks
The integration of Convolutional Neural Networks (CNNs) in seismic interpretation represents a significant advancement in harnessing deep learning capabilities to improve data processing accuracy and efficiency. CNNs excel in capturing spatial dependencies within seismic images, allowing for feature extraction and pattern recognition with remarkable precision. The key characteristic of CNNs lies in their hierarchical architecture that automatically learns features directly from seismic data, making them a popular choice in image-based applications. Within this article, CNNs play a crucial role in enhancing subsurface imaging by identifying complex geological features and anomalies, providing geoscientists with detailed insights for accurate interpretation and decision-making. While CNNs offer unparalleled feature learning capabilities, challenges related to model complexity and computational resources should be carefully managed to maximize their benefits in seismic interpretation.
Recurrent Neural Networks
The incorporation of Recurrent Neural Networks (RNNs) in seismic interpretation introduces a dynamic approach to analyzing temporal dependencies and sequential data patterns inherent in seismic datasets. RNNs excel in capturing historical information and contextual relationships across sequential data points, making them highly effective in time-series analysis and sequence prediction tasks. The key characteristic of RNNs lies in their ability to retain long-term memory and feedback loops, enabling them to model intricate temporal relationships within seismic data. In this article, RNNs enhance the understanding of seismic signals by uncovering time-dependent features and trends, facilitating more accurate predictions and interpretations of subsurface characteristics. Despite their strengths in modeling sequential data, challenges such as vanishing gradients and computational inefficiencies must be addressed to leverage the full potential of RNNs in seismic data processing.
Integration and Visualization
In the realm of seismic data processing for precision and efficiency, the crucial phase of Integration and Visualization plays an indispensable role. Integration involves the amalgamation of various datasets and information sources to create a comprehensive view of the subsurface structures. This process is vital as it enables the interpreter to gain a holistic understanding of the geological features being studied. Moreover, Visualization is key in portraying complex data in a manner that is easy to interpret and analyze. By utilizing advanced graphical tools and software, seismic interpreters can translate raw data into meaningful visual representations, aiding in the extraction of valuable insights.
Data Integration
Multidisciplinary Data Fusion
Multidisciplinary Data Fusion is a specific facet of data integration that involves merging information from diverse disciplines such as geophysics, geology, and engineering. This integration approach enhances the accuracy and completeness of the subsurface models by incorporating insights from various fields. The key characteristic of Multidisciplinary Data Fusion lies in its ability to provide a multi-faceted perspective, allowing for a more robust interpretation of seismic data. It is a favored choice in this article due to its holistic nature, which aligns with the objective of achieving precision and efficiency in seismic data processing. The unique feature of Multidisciplinary Data Fusion is its capacity to mitigate interpretation biases and improve the reliability of the final subsurface image. However, challenges may arise in reconciling conflicting information from different disciplines, necessitating a thoughtful approach to data integration.
Seismic-Well Calibration
Seismic-Well Calibration is another significant aspect essential for achieving accuracy in seismic data interpretation. This process involves aligning seismic data with information obtained from wells drilled into the subsurface. By calibrating seismic responses with well data, interpreters can validate the geological features identified through seismic imaging. The key characteristic of Seismic-Well Calibration is its ability to provide ground truth data for validating seismic interpretations, thereby enhancing the reliability of the subsurface model. This technique is a valuable choice in this article as it bridges the gap between seismic observations and actual subsurface properties. The unique feature of Seismic-Well Calibration is its capability to refine seismic interpretation, leading to more precise imaging and improved subsurface understanding. However, challenges may arise in accurately correlating seismic responses with well data due to inherent uncertainties in both datasets.
Geo-Modeling Software
3D Visualization Tools
3D Visualization Tools are instrumental in displaying seismic data in a three-dimensional space, allowing interpreters to visualize subsurface structures with depth perception. The key characteristic of 3D Visualization Tools is their ability to present complex data in an intuitive and interactive manner, enhancing the interpretability of seismic images. This choice is favored in the article for its capacity to provide advanced visualization capabilities that aid in comprehensive subsurface analysis. The unique feature of 3D Visualization Tools is their potential to simulate real-world geological scenarios, facilitating a comprehensive understanding of the subsurface environment. Advantages of these tools include improved spatial awareness and enhanced interpretation accuracy, although challenges may arise in processing large datasets and maintaining real-time rendering performance.
Spectral Decomposition Software
Spectral Decomposition Software plays a vital role in identifying and analyzing frequency components within seismic data, allowing interpreters to extract valuable information related to subsurface properties. The key characteristic of Spectral Decomposition Software is its capability to decompose seismic signals into various frequency bands, enabling interpreters to assess reservoir characteristics and fluid properties. This software is a beneficial choice in the article for its ability to enhance frequency content analysis and attribute mapping for subsurface reservoirs. The unique feature of Spectral Decomposition Software is its capacity to improve the resolution of seismic attributes, providing detailed insights into subsurface structures. Advantages of this software include enhanced reservoir characterization and increased precision in seismic interpretation, although challenges may arise in setting suitable parameters for spectral decomposition and interpreting complex frequency results.
Conclusion
Future Trends
Automation in Seismic Interpretation
Enhanced Data Analytics
Enhanced data analytics represents a paradigm shift in deriving meaningful insights from seismic data. By employing advanced analytical techniques such as machine learning, pattern recognition, and predictive modeling, enhanced data analytics enables in-depth data interpretation, trend identification, and anomaly detection. The key characteristic of enhanced data analytics lies in its ability to uncover hidden patterns, correlations, and anomalies that may not be apparent through traditional methods. While it offers enhanced decision-making capabilities and predictive modeling accuracy, careful consideration of data preprocessing, model selection, and interpretational limitations is essential to maximize the efficacy of enhanced data analytics in seismic data processing.