Mastering Business Analytics: A Comprehensive Guide for Strategic Decision-Making
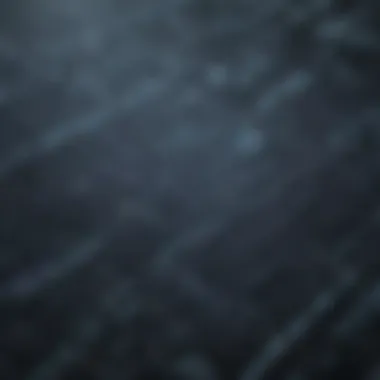
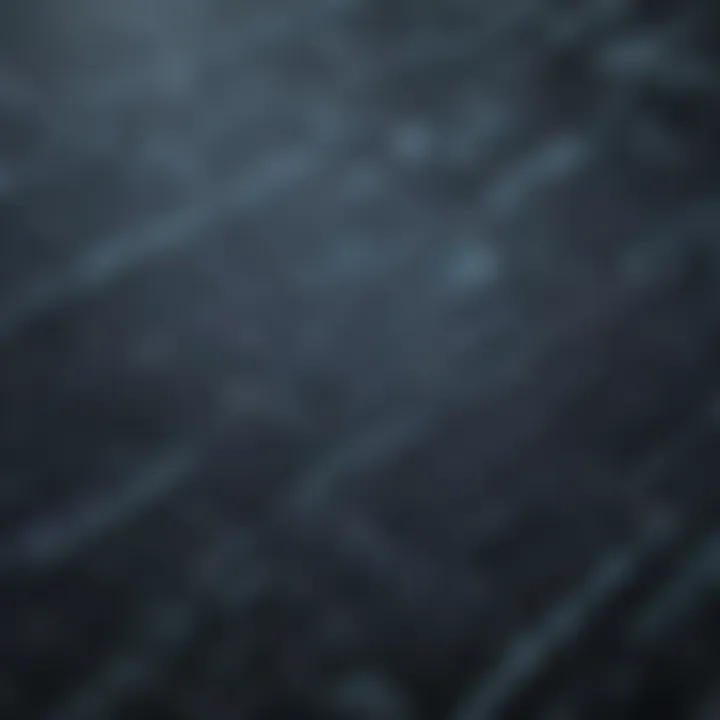
Overview of Business Analytics
Business analytics is a crucial aspect of today's data-driven business landscape, playing a vital role in strategic decision-making and organizational growth. Understanding the fundamentals of business analytics is paramount for professionals looking to harness the power of data effectively. Exploring key concepts, methodologies, tools, and applications in business analytics provides valuable insights for individuals aiming to make informed decisions based on data-driven insights. With the rapid advancement of technology, the significance of business analytics in the tech industry continues to grow, making it imperative for organizations to leverage data for a competitive edge.
Fundamentals of Business Analytics
To delve deeper into business analytics, it is essential to grasp the core principles and theories that underpin this field. Key terminology and definitions such as predictive analytics, data mining, and machine learning form the bedrock of business analytics knowledge. Understanding these basic concepts is foundational for professionals seeking to utilize data for actionable insights. The history and evolution of business analytics reveal its transformation from a basic reporting tool to a sophisticated decision-making asset, reflecting the evolution of technology in interpreting and analyzing data.
Practical Applications in Business Analytics
Real-world case studies and applications demonstrate the tangible value of business analytics in various industries. Examining hands-on projects and demonstrations showcases the practicality and efficacy of implementing analytics to drive business growth. Code snippets and implementation guidelines offer a glimpse into the technical aspect of utilizing analytics tools effectively. By focusing on applications, professionals can witness how data translates into actionable strategies and measurable outcomes.
Advanced Topics and Current Trends in Business Analytics
Remaining at the forefront of business analytics requires an understanding of advanced techniques and cutting-edge developments. Exploration of the latest trends in the field, such as big data analytics, artificial intelligence, and prescriptive analytics, paves the way for future innovation and growth. By delving into advanced methodologies, professionals can gain insight into the intricate processes of data analysis and decision-making. Understanding future prospects and upcoming trends in business analytics enables organizations to proactively adapt to changing market dynamics and consumer demands.
Tips and Resources for Continued Learning
For individuals looking to deepen their understanding of business analytics, recommended books, courses, and online resources serve as valuable tools for further learning. Leveraging software and tools for practical usage enhances the application of theoretical knowledge in real-world scenarios. By equipping oneself with the right resources, professionals can stay abreast of the evolving landscape of business analytics and continuously enhance their skills in data-driven decision-making.
In the realm of modern business, the strategic implementation and understanding of business analytics play a pivotal role in shaping organizational success and growth. This article serves as a comprehensive guide, delving deep into the various facets of business analytics, from fundamental concepts to advanced analytical methods, providing insightful perspectives for professionals and enthusiasts aiming to harness the power of data for informed decision-making and business enhancement.
Understanding the Basics
Definition of Business Analytics
Business analytics refers to the iterative exploration of organization's data to drive informative insights for effective decision-making. It involves the utilization of statistical analysis, predictive modeling, and data optimization techniques to uncover patterns and trends within the data landscape. The key characteristic of business analytics lies in its ability to transform raw data into actionable intelligence, aiding businesses in identifying opportunities, mitigating risks, and enhancing operational efficiency. While its advantages are manifold, such as enabling data-driven strategies, it also poses challenges like complexity in data interpretation and resource-intensive implementation.
Importance in Modern Business
In today's data-centric business environment, the importance of business analytics cannot be overstated. It serves as the cornerstone for organizational success by providing valuable insights into market trends, consumer behavior, and internal operations. The key characteristic of business analytics in modern business lies in its capacity to align decision-making processes with empirical evidence, fostering a more accurate and strategic approach to problem-solving. While its advantages include improved productivity and competitive edge, potential drawbacks may include over-reliance on data without holistic consideration of external factors.
Role in Decision-Making
The role of business analytics in decision-making revolves around facilitating informed and strategic choices based on data-driven evidence. It empowers organizations to optimize their operational processes, identify growth opportunities, and address potential threats through a systematic analysis of internal and external data sources. The key characteristic of business analytics in decision-making is its ability to enhance the quality and precision of strategic decisions by reducing uncertainty and cognitive biases. While its advantages include improved risk management and performance forecasting, challenges may arise concerning data accuracy and the interpretation of complex analytical models.
Evolution and Trends
Historical Development
The historical development of business analytics traces back to the early foundations of statistical analysis and operations research, evolving into sophisticated data mining and predictive modeling techniques. Over the years, advancements in technology have propelled the field towards greater automation, scalability, and real-time analytics capabilities. The key characteristic of historical development in business analytics lies in its iterative progression towards more efficient and insightful data analysis methodologies. While its advantages include enhanced decision-making processes and improved resource allocation, potential disadvantages may involve steep learning curves and legacy system integration challenges.
Current Trends in Business Analytics
The current trends in business analytics reflect a shift towards more agile, adaptive, and AI-driven approaches to data analysis. Concepts such as machine learning, natural language processing, and big data analytics have gained prominence, enabling organizations to extract deeper meanings from complex datasets. The key characteristic of current trends in business analytics is their focus on real-time insights, predictive modeling, and prescriptive optimization techniques. While their advantages encompass increased operational efficiency and proactive decision-making, potential challenges may arise in data governance and model interpretability.
Scope and Applications
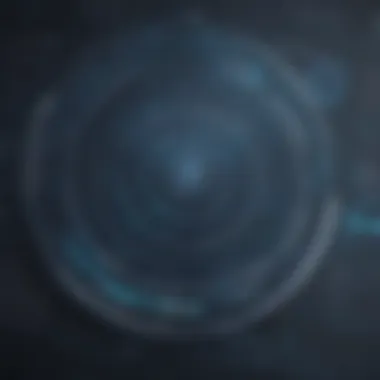
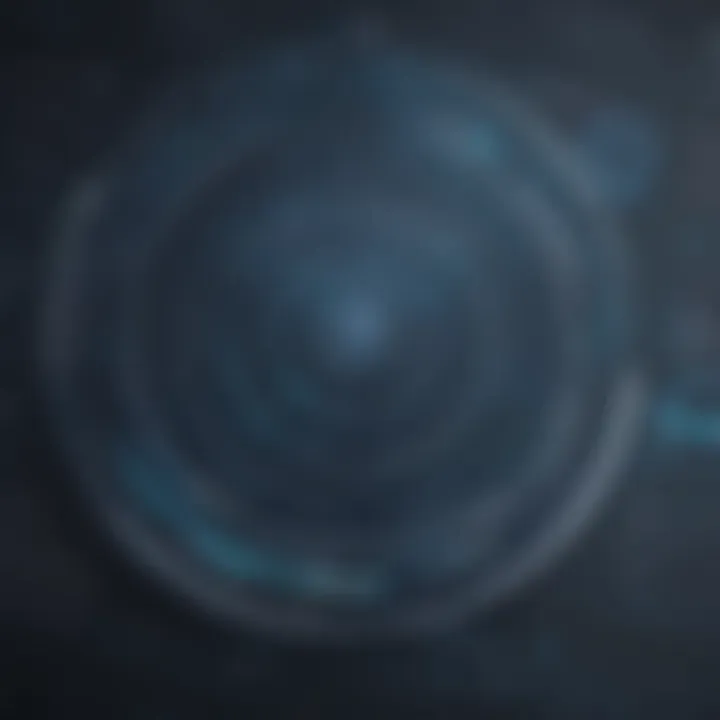
Marketing Analytics
Marketing analytics entails the utilization of data-driven strategies to optimize marketing campaigns, target audience segmentation, and customer engagement initiatives. It enables organizations to measure the effectiveness of their marketing efforts, track consumer behavior patterns, and adapt to dynamic market trends. The key characteristic of marketing analytics lies in its ability to identify actionable insights for personalized marketing strategies and revenue growth. While its advantages encompass improved ROI and customer retention, challenges may surface concerning data privacy regulations and ethical data usage.
Financial Analytics
Financial analytics involve the application of quantitative methods to interpret financial data, derive performance metrics, and assess investment risks. It enables organizations to make informed financial decisions, allocate resources efficiently, and forecast future financial outcomes. The key characteristic of financial analytics is its capacity to provide actionable insights for budgeting, financial planning, and risk management. While its advantages encompass improved financial health and strategic investment planning, potential disadvantages may include algorithmic biases and data security vulnerabilities.
Operations Analytics
Operations analytics focus on enhancing operational efficiency, streamlining supply chain processes, and optimizing resource utilization through data-driven insights. It enables organizations to identify bottlenecks, reduce wastage, and improve production workflows for cost savings and productivity gains. The key characteristic of operations analytics lies in its ability to leverage real-time data for continuous process improvement and sustainable operational practices. While its advantages include enhanced quality control and asset utilization, challenges may arise in data integration and operational scalability.
Risk Analytics
Risk analytics involve the identification, assessment, and mitigation of potential risks that could impact organizational objectives and performance. It employs predictive modeling, scenario analysis, and risk assessments to assess vulnerabilities, forecast outcomes, and develop risk mitigation strategies. The key characteristic of risk analytics is its proactive approach to risk management, enabling organizations to anticipate and prevent potential threats before they materialize. While its advantages encompass improved disaster recovery and regulatory compliance, potential challenges may include model accuracy issues and data silo challenges.
Tools and Technologies
Exploring Business Analytics Techniques
In the realm of business analytics, understanding and implementing various techniques play a critical role in transforming raw data into actionable insights. Exploring Business Analytics Techniques is pivotal in this comprehensive guide as it encompasses a range of methodologies that aid in decision-making and enhancing business strategies. By delving into descriptive, predictive, prescriptive analytics, and advanced analytical methods, professionals can leverage these techniques to derive valuable insights and make data-driven decisions to drive business growth and success.
Descriptive Analytics
Analyzing Historical Data
Analyzing Historical Data holds immense importance within the scope of business analytics as it allows professionals to examine past trends, patterns, and behaviors to gain a deeper understanding of business performance. By dissecting historical data, organizations can identify key indicators, anomalies, and correlations that can inform future decision-making processes. This analytical process provides a foundation for predictive and prescriptive analytics, enabling businesses to make informed strategic choices based on historical evidence and trends. The unique feature of Analyzing Historical Data lies in its ability to uncover hidden insights within historical datasets, empowering organizations to proactively address challenges and capitalize on opportunities that align with business objectives.
Dashboard Reporting
Dashboard Reporting stands out as a vital component in business analytics, offering stakeholders a visual representation of key performance metrics and KPIs for effective decision-making. By consolidating complex data into intuitive and interactive dashboards, organizations can track progress, identify trends, and communicate insights with stakeholders in a simplified format. The inherent value of Dashboard Reporting lies in its capacity to provide real-time updates, actionable insights, and customizable views that cater to the specific needs of different user groups. While Dashboard Reporting streamlines data visualization and interpretation processes, it also presents challenges in ensuring data accuracy, relevance, and alignment with business goals.
Predictive Analytics
Forecasting Future Trends
Forecasting Future Trends is a fundamental aspect of predictive analytics that enables organizations to anticipate market shifts, consumer behavior, and emerging opportunities. By applying statistical models, forecasting techniques, and trend analysis to historical data, businesses can make data-driven predictions that guide strategic planning and resource allocation. The significance of Forecasting Future Trends lies in its ability to reduce uncertainty, mitigate risks, and capitalize on emerging market trends, giving organizations a competitive edge in dynamic industries. While Forecasting Future Trends provides valuable predictive insights, it also requires continuous validation, refinement, and adaptation to align with changing market dynamics.
Regression Analysis
Regression Analysis serves as a cornerstone in predictive analytics, allowing organizations to establish relationships between variables, predict outcomes, and identify influential factors affecting business performance. By examining historical data patterns and correlations, regression analysis facilitates the development of predictive models that guide decision-making processes. The key characteristic of Regression Analysis lies in its versatility, as it can be applied across various industry domains to forecast trends, insights, and outcomes based on historical data patterns. While Regression Analysis offers valuable predictive capabilities, it also poses challenges in ensuring model accuracy, statistical significance, and data interpretation that drive actionable business recommendations.
Prescriptive Analytics
Optimizing Decision-Making
Optimizing Decision-Making represents a critical component of prescriptive analytics, enabling organizations to streamline processes, enhance efficiencies, and maximize outcomes through data-driven strategies. By synthesizing vast amounts of data, prescriptive analytics empowers decision-makers to evaluate multiple scenarios, optimize resource allocation, and minimize risks while maximizing returns. The key characteristic of Optimizing Decision-Making lies in its ability to recommend the best course of action based on data-driven insights, predictive modeling, and advanced algorithms. While prescriptive analytics offers invaluable decision-making support, it requires continuous monitoring, validation, and adaptation to ensure alignment with evolving business objectives.
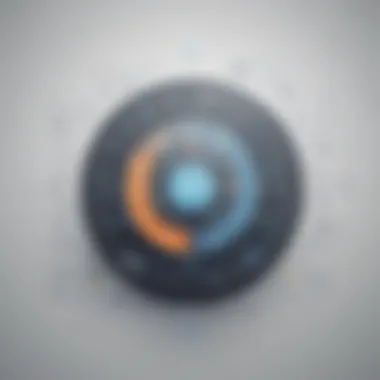
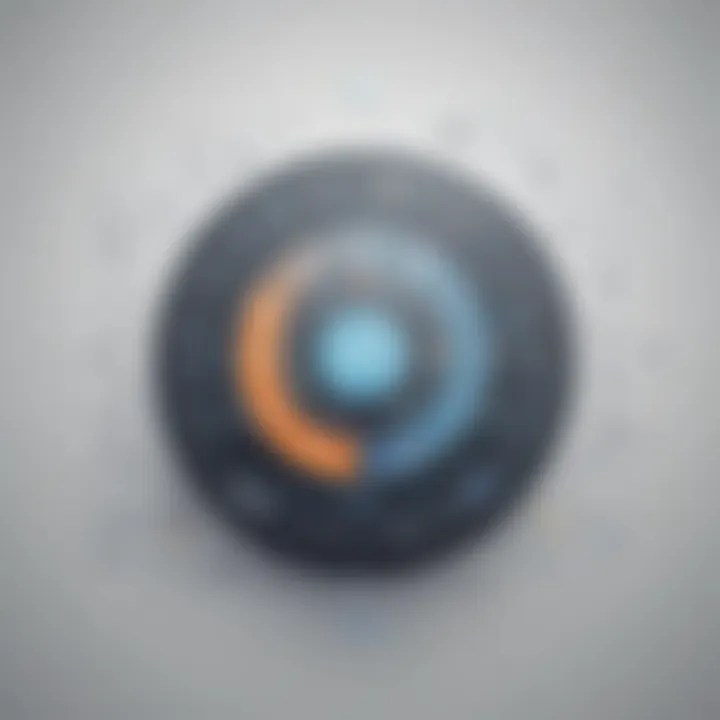
Simulation Techniques
Simulation Techniques play a pivotal role in prescriptive analytics, allowing organizations to simulate complex scenarios, test hypotheses, and evaluate potential outcomes in a controlled environment. By creating virtual models based on historical data and dynamic variables, simulation techniques provide decision-makers with actionable insights, risk analysis, and strategic recommendations. The unique feature of Simulation Techniques lies in their ability to assess multiple decision pathways, scenarios, and constraints to identify optimal strategies that align with organizational goals. While Simulation Techniques offer valuable predictive capabilities, they require robust modeling, accurate data inputs, and thorough scenario planning to deliver meaningful and actionable insights.
Advanced Analytical Methods
Machine Learning Algorithms
Machine Learning Algorithms stand out as advanced analytical methods that leverage algorithms, models, and data patterns to make intelligent predictions, automate processes, and enhance decision-making in real-time. By training algorithms on historical data sets, machine learning enables organizations to uncover complex relationships, predict trends, and optimize operations. The key characteristic of Machine Learning Algorithms lies in their adaptability, scalability, and versatility across diverse industry applications, from healthcare to finance. While machine learning algorithms offer unprecedented predictive power, they require extensive data sets, algorithm training, and ongoing optimization to deliver accurate and actionable insights.
Natural Language Processing
Natural Language Processing emerges as a cutting-edge analytical method that interprets and generates human language to extract insights, sentiment analysis, and data categorization from unstructured text data. By processing and analyzing vast amounts of textual data, natural language processing enables organizations to derive valuable insights, automate customer interactions, and enhance data-driven decision-making processes. The unique feature of Natural Language Processing lies in its ability to understand context, language nuances, and sentiment variations, empowering organizations to extract meaningful information from textual sources. While natural language processing offers advanced analytical capabilities, it requires robust algorithms, language models, and ongoing refinement to ensure accuracy, relevancy, and compliance with data privacy regulations.
Deep Learning
Deep Learning stands at the forefront of advanced analytical methods, utilizing neural networks, hierarchical learning, and complex algorithms to mimic the human brain's ability to recognize patterns, process information, and make decisions. By deploying deep learning models on vast data sets, organizations can achieve unparalleled accuracy, predictive power, and innovative solutions in diverse domains. The key characteristic of Deep Learning lies in its ability to handle unstructured data, hierarchical patterns, and multi-dimensional relationships, enabling organizations to unlock insights, automate processes, and drive innovation. While deep learning offers transformative analytical capabilities, it requires substantial computational resources, model training, and validation to unleash its full potential and deliver actionable business outcomes.
Implementing Business Analytics Strategies
Implementing Business Analytics Strategies plays a crucial role in maximizing the advantages of business analytics in modern business operations. It involves a systematic approach to collecting, preparing, visualizing, interpreting data, and building predictive models for effective decision-making. By focusing on this aspect, businesses can harness the power of analytics to enhance performance, streamline processes, and gain a competitive edge in the market.
Data Collection and Preparation
Data Integration
Data Integration is a fundamental aspect of Implementing Business Analytics Strategies as it involves combining data from various sources to provide a unified view. The key characteristic of Data Integration lies in its ability to aggregate information from disparate systems, databases, and applications into a centralized repository. This centralized data hub facilitates seamless analysis and reporting processes, enabling organizations to derive valuable insights for informed decision-making. Despite its advantages in creating a holistic view of data, Data Integration may pose challenges related to data consistency, compatibility, and scalability.
Data Cleaning
On the other hand, Data Cleaning focuses on refining the quality of data by identifying and rectifying inconsistencies, errors, and redundancies. This process is vital for ensuring the accuracy and reliability of data used for analysis and model development. The primary characteristic of Data Cleaning is its role in enhancing data integrity and reducing the risk of misleading conclusions. While Data Cleaning enhances the overall data quality, it can be a time-consuming task, requiring diligent attention to detail and thorough validation procedures.
Data Visualization and Interpretation
Dashboard Design
Effective Dashboard Design is instrumental in transforming complex data sets into visually appealing and easily understandable representations. The key characteristic of Dashboard Design lies in its ability to present critical data metrics, trends, and insights in a concise and user-friendly format. Dashboards offer decision-makers a real-time snapshot of key performance indicators, enabling swift and data-driven decision-making. However, the design process should balance aesthetics with functionality to ensure optimal user engagement and comprehension.
Insight Extraction
Insight Extraction involves the extraction of meaningful patterns, trends, and correlations from data to derive actionable insights. The key characteristic of Insight Extraction is its capacity to turn raw data into valuable information that guides strategic decision-making. By employing advanced analytical techniques, organizations can uncover hidden opportunities, risks, and potential areas for optimization. While Insight Extraction enhances decision-making capabilities, organizations must validate and contextualize extracted insights to ensure their relevance and applicability.
Building Predictive Models
Model Development
Model Development is a critical step in leveraging predictive analytics to forecast trends, outcomes, and future scenarios. The key characteristic of Model Development is its iterative process of constructing, testing, and refining predictive models based on historical data. This iterative approach allows organizations to enhance the accuracy and reliability of predictions, enabling proactive decision-making strategies. However, Model Development requires domain expertise, robust validation methods, and continuous optimization to maximize predictive accuracy and usability.
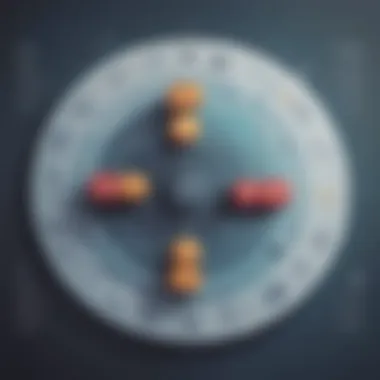
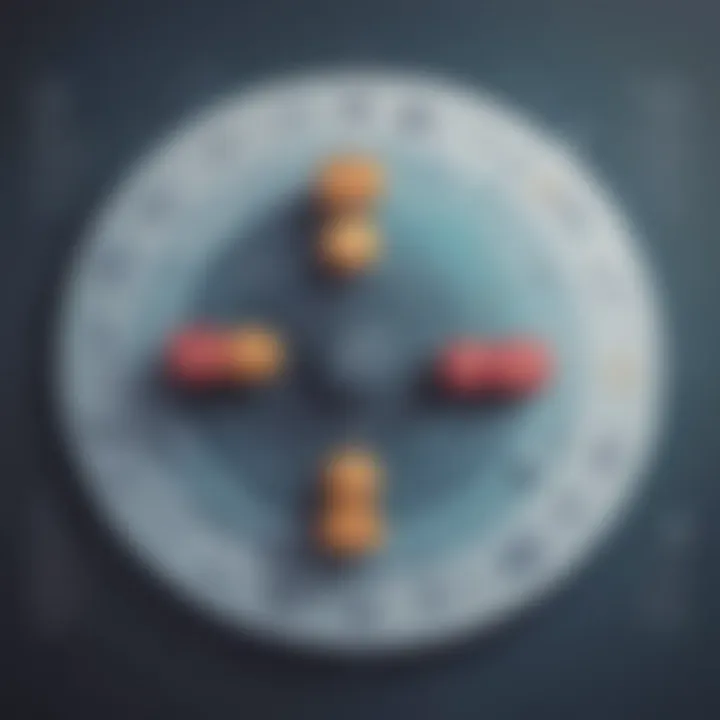
Validation Techniques
Validation Techniques are essential in assessing the performance and reliability of predictive models in real-world scenarios. The key characteristic of Validation Techniques lies in their ability to evaluate model accuracy, generalizability, and predictive power using validation data sets. By employing diverse validation methods such as cross-validation, holdout validation, and AB testing, organizations can ensure the robustness and effectiveness of predictive models. However, selecting an appropriate validation technique is critical to avoiding overfitting or bias in model evaluation processes.
Deployment and Monitoring
Integration with Business Processes
Integrating predictive models with existing business processes is vital for translating analytical insights into actionable strategies. The key characteristic of Integration with Business Processes is its alignment with organizational goals, workflows, and decision-making frameworks. By seamlessly embedding predictive models into operational workflows, organizations can enhance process efficiency, optimize resource allocation, and drive consistent performance improvements. However, effective integration requires continuous monitoring, feedback integration, and adaptation to evolving business needs.
Performance Tracking
Performance Tracking involves monitoring and evaluating the efficacy and impact of predictive models on business performance. The key characteristic of Performance Tracking is its focus on assessing key performance metrics, variances, and outcomes resulting from model deployment. By tracking performance indicators such as accuracy rates, error margins, and ROI metrics, organizations can measure the success of predictive models and identify areas for improvement. However, continuous performance tracking demands ongoing analysis, iterative refinement, and strategic recalibration to maintain model effectiveness and relevance.
Future Trends in Business Analytics
In the fast-paced world of business analytics, staying abreast of future trends is paramount for professionals seeking to gain a competitive edge. Future Trends in Business Analytics encompasses the evolving landscape of technologies, methodologies, and applications that are shaping the industry's trajectory. Understanding these trends offers a strategic advantage in harnessing data for informed decision-making and sustainable business growth.
Emerging Technologies
AI and Machine Learning
AI and Machine Learning revolutionize data analysis by automating pattern recognition and predictive modeling. These technologies streamline decision-making processes, enhance data accuracy, and uncover valuable insights from complex datasets. Their adaptability and scalability make them indispensable tools for businesses aiming to optimize operations and drive innovation.
Big Data Analytics
Big Data Analytics empowers organizations to process vast and varied data sources efficiently. By leveraging advanced algorithms and data processing techniques, Big Data Analytics uncovers hidden patterns, trends, and correlations within data sets. This capability enables businesses to make data-driven decisions swiftly and strategically.
IoT Integration
IoT Integration connects devices and systems to gather real-time data for analysis. This interconnectivity enhances operational efficiency, enables predictive maintenance, and facilitates seamless communication between devices. Leveraging IoT Integration in business analytics allows for holistic insights into processes, performance optimization, and proactive decision-making.
Industry Applications
Healthcare
Healthcare analytics plays a pivotal role in improving patient care, optimizing hospital operations, and facilitating medical research. By harnessing data from electronic health records, medical imaging, and wearable devices, healthcare professionals can enhance diagnosis accuracy, treatment effectiveness, and operational efficiency.
E-Commerce
E-Commerce analytics drives customer segmentation, personalized marketing strategies, and product recommendations. Analyzing user behavior, purchasing patterns, and market trends enables E-Commerce businesses to tailor their offerings, improve user experience, and optimize sales conversion rates.
Supply Chain Management
Supply Chain Management benefits from analytics by optimizing inventory management, demand forecasting, and logistics operations. Analyzing supply chain data enhances transparency, cost efficiency, and responsiveness to market fluctuations, mitigating risks and improving overall operational resilience.
Ethical Considerations
Privacy Concerns
Privacy concerns in business analytics revolve around data protection, consent management, and information security. Safeguarding sensitive data, ensuring compliance with regulations, and respecting user privacy rights are paramount in ethical data practices. Addressing privacy concerns builds trust with customers and fortifies the organizational reputation.
Bias and Fairness
Ensuring bias mitigation and fairness in data analysis is essential for ethical decision-making and societal impact. Detecting and rectifying biases in algorithms, datasets, and decision models promotes equity and inclusivity. Emphasizing bias awareness and fairness standards fosters transparency and accountability in business analytics practices.