Unlocking Data Analyst KPI Mastery: Examples & Insights Revealed
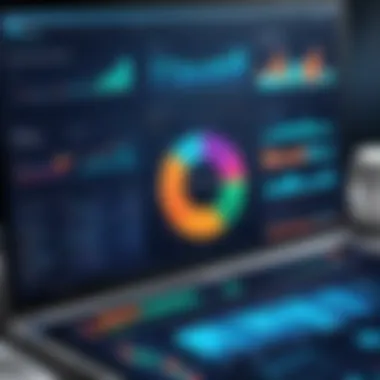
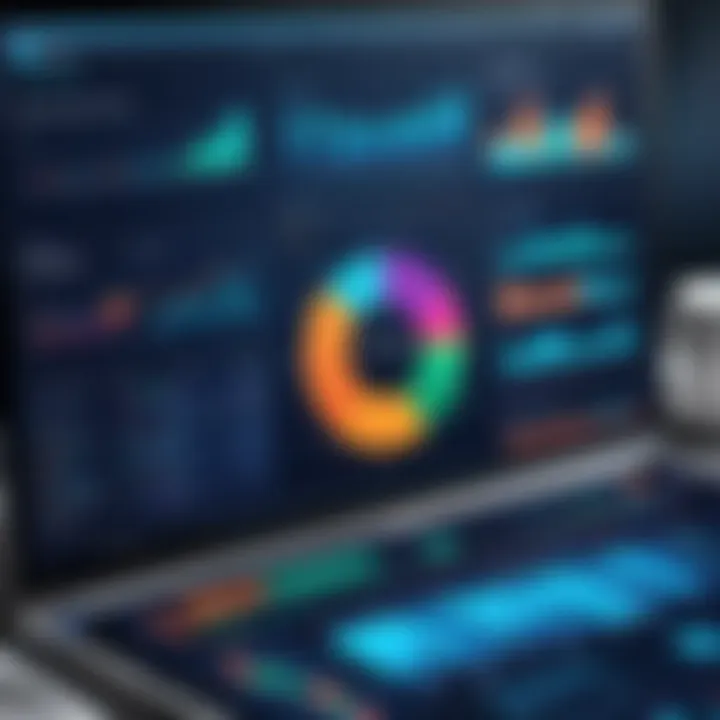
Overview of Topic
Data analysts play a pivotal role in today's data-driven world by extracting insights from complex data sets. Understanding key Performance Indicators (KPIs) is crucial for their success. By delving into various KPI examples, data analysts can effectively measure their performance and contribute to informed decision-making processes.
Fundamentals Explained
In the realm of data analysis, it is essential to grasp the core principles and theories underlying KPIs. Key terminology such as metrics, targets, and benchmarks hold significant importance. Data analysts must master basic concepts to interpret KPIs accurately and derive meaningful conclusions from data.
Practical Applications and Examples
Real-world case studies serve as invaluable learning tools for data analysts. By exploring hands-on projects and code snippets, analysts can gain practical insights into implementing KPIs effectively. Demonstrating the application of KPIs in various scenarios enhances their analytical skills and decision-making abilities.
Advanced Topics and Latest Trends
As technology evolves, data analysis techniques advance rapidly. Data analysts must stay abreast of cutting-edge developments to remain competitive in the field. Exploring advanced methodologies and future prospects equips analysts with the knowledge needed to excel in their roles and adapt to emerging trends.
Tips and Resources for Further Learning
To deepen their understanding of Data Analyst KPIs, individuals can explore recommended books, online courses, and tools tailored for practical usage. Leveraging resources like specialized software enhances analytical capabilities and fosters continuous learning and growth.
Prelude
Understanding Data Analyst KPIs
Importance of KPIs in Data Analysis
Engaging in the meticulous evaluation of Key Performance Indicators (KPIs) is pivotal in the domain of data analysis. Acknowledging the fulcrum that KPIs represent in deciphering complex datasets can potentially revolutionize strategic decision-making processes. The salient characteristic of leveraging KPIs in data analysis lies in their ability to distill voluminous data points into digestible insights, thus facilitating data-driven strategies. The unique attribute of KPIs in data analysis manifests in their capacity to quantify intangible variables, adding a layer of precision to decision-making processes.
Purpose of Setting KPIs
The essence of setting Key Performance Indicators (KPIs) transcends mere data quantification; it embodies a strategic blueprint outlining organizational objectives. Setting KPIs provides a tangible roadmap for data analysts, aligning their efforts with overarching business goals. The significance of KPI setting lies in its role as a navigational tool, directing data analysis towards delineated targets. Embracing the practice of setting KPIs not only streamlines analytical endeavors but also fosters a symbiotic relationship between data analysis and strategic alignment.
Significance of Effective KPIs
Benefits of Utilizing KPIs
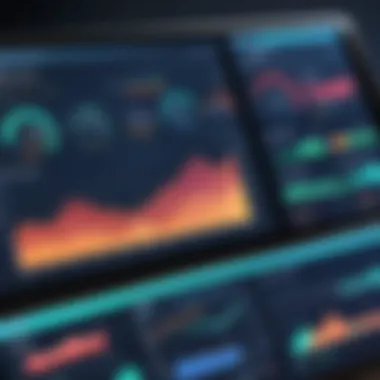
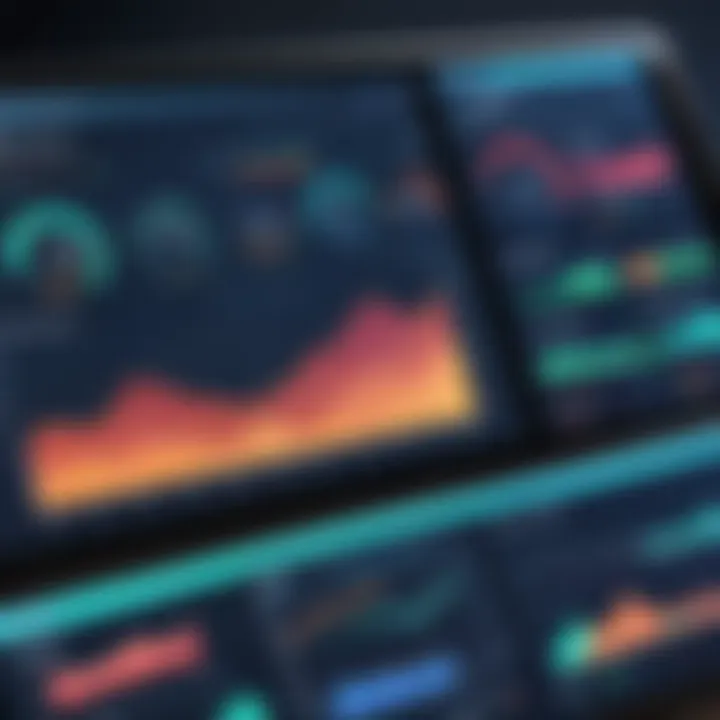
Harnessing the power of Key Performance Indicators (KPIs) yields multifaceted benefits in the landscape of data analysis. The efficacy of utilizing KPIs resonates in their ability to quantify intangible metrics, offering a tangible yardstick for performance evaluation. Leveraging KPIs not only enhances data analysis precision but also fosters a culture of continuous improvement, propelling organizational growth. The distinct advantage of KPI utilization lies in its transformative impact on data analysis methodologies, catalyzing data-driven decision-making processes.
Impact on Decision-Making
The reverberations of effective Key Performance Indicators (KPIs) are most pronounced in the sphere of decision-making. KPIs serve as decision-enablers, providing data analysts with crisp insights to steer strategic initiatives. The pivotal role of KPIs in decision-making manifests in their capacity to transform raw data into actionable intelligence, arming decision-makers with empirical evidence. Integrating KPIs into decision-making processes not only enhances efficiency but also fortifies organizational agility in navigating dynamic business landscapes and exigent scenarios.
Common KPI Examples for Data Analysts
In the realm of data analysis, understanding and implementing key performance indicators (KPIs) play a pivotal role in assessing the effectiveness and efficiency of analytical processes. For data analysts, common KPI examples serve as essential tools to gauge performance levels and monitor progress accurately. By delving into metrics such as data quality, performance-based indicators, and business impact measures, analysts can derive valuable insights that drive strategic decision-making. These KPI examples not only provide quantifiable data points but also offer a structured approach in evaluating the success and impact of analytical activities.
Data Quality Metrics
Data quality stands as a cornerstone in data analysis, emphasizing the accuracy, consistency, and reliability of datasets. Within this domain, metrics like Accuracy Rate and Completeness Level hold significant importance in identifying the precision and thoroughness of analytical outputs. The Accuracy Rate metric measures the extent to which data points align with the actual information, ensuring minimal errors and discrepancies. On the other hand, the Completeness Level metric focuses on the entirety of data available for analysis, indicating the sufficiency of information for making informed decisions. Both these metrics contribute to enhancing the overall data quality and reliability, enabling analysts to draw meaningful conclusions and insights from their analyses.
Accuracy Rate
Accuracy Rate, a fundamental metric in data quality assessment, signifies the degree of correctness in data interpretation. By evaluating the accuracy rate, analysts can validate the authenticity of their findings and minimize the risk of erroneous insights. Its distinct feature lies in its ability to pinpoint discrepancies and inconsistencies within datasets, enabling analysts to rectify errors and maintain data integrity. While Accuracy Rate serves as a dependable indicator of data precision, it may also pose challenges in capturing nuanced inaccuracies or outliers, necessitating supplementary validation methods to ensure comprehensive data accuracy.
Completeness Level
Completeness Level, another pivotal metric in data quality evaluation, showcases the extent of data availability and comprehensiveness within analytical datasets. This metric highlights the thoroughness of data collection and consolidation, ensuring that analysts have access to sufficient information for robust analysis. Its unique feature lies in its capacity to reveal gaps or deficiencies in data coverage, prompting analysts to seek additional sources or refine data collection processes to achieve comprehensive insights. While Completeness Level enhances the depth of analysis, it may encounter limitations in assessing data relevancy or timeliness, requiring analysts to balance quantity with quality in data integration.
Performance-based KPIs
Performance-based KPIs offer valuable insights into the operational efficiency and effectiveness of data analysis processes. Metrics such as Throughput and Efficiency Ratio enable analysts to measure the speed, productivity, and resource utilization in analytical tasks, optimizing workflow performance and output quality. These KPIs not only facilitate performance monitoring but also support continuous improvement initiatives by identifying bottlenecks, enhancing resource allocation, and streamlining operational processes.
Throughput
Throughput, a key performance metric in data analysis, signifies the rate of data processing or task completion within a specified timeframe. By assessing throughput levels, analysts can evaluate the efficiency and productivity of analytical workflows, identifying areas for optimization and enhancement. Its critical characteristic lies in its capacity to quantify the output speed and volume of analytical operations, facilitating streamlined decision-making and resource planning. While Throughput serves as an essential indicator of operational efficiency, it may encounter challenges in capturing qualitative aspects of analysis, prompting analysts to combine throughput data with qualitative assessments for comprehensive performance evaluation.
Efficiency Ratio
Efficiency Ratio, an essential performance metric in data analysis, reflects the ratio of output generated to the resources invested in analytical activities. This metric underscores the cost-effectiveness and resource efficiency of analytical procedures, guiding analysts in optimizing resource allocation and maximizing output value. Its distinctive feature lies in its ability to measure the output-to-input relationship, highlighting areas of inefficiency or productivity gaps within analytical workflows. While Efficiency Ratio provides valuable insights into resource utilization, it may require detailed input/output categorization to ensure accurate performance assessment, emphasizing the need for transparent and standardized efficiency measurements.
Business Impact Indicators
Business impact indicators elucidate the tangible outcomes and implications of analytical insights on organizational performance and strategic decision-making. Metrics like Revenue Growth and Customer Retention Rate offer valuable perspectives on the financial, operational, and customer relationship aspects influenced by analytical activities. By tracking these indicators, analysts can align analytical efforts with business objectives, drive revenue growth, and foster customer loyalty through data-driven strategies.
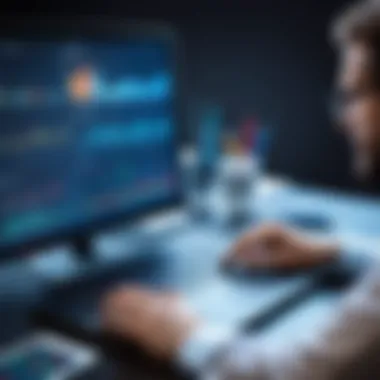
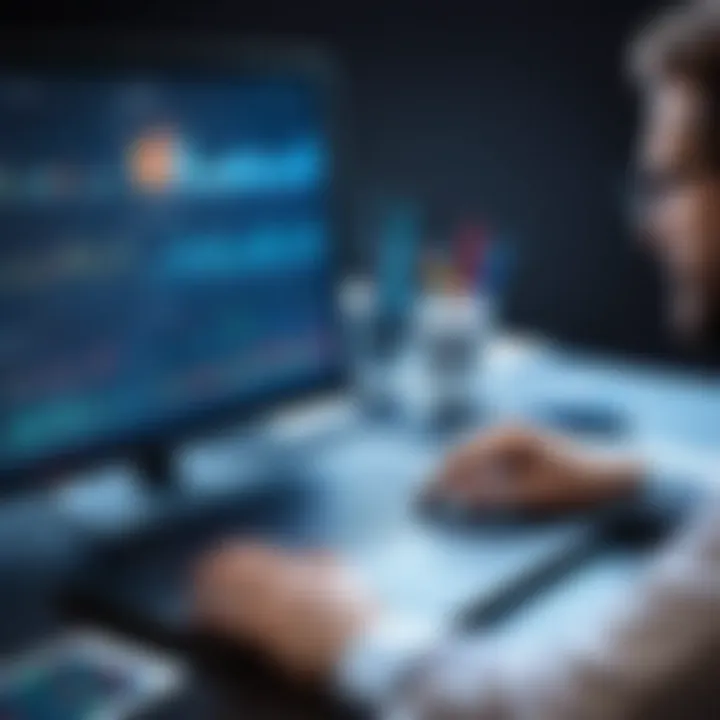
Revenue Growth
Revenue Growth, a critical business indicator in data analysis, signifies the percentage increase in company revenue over a specific period. By analyzing revenue growth trends, analysts can assess the effectiveness of sales strategies, marketing campaigns, and operational initiatives in driving financial performance. Its key characteristic lies in its capacity to reflect the financial health and growth trajectory of a business, guiding strategic decisions and investment planning. While Revenue Growth serves as a strategic performance indicator, it may face challenges in isolating the impact of individual factors on revenue fluctuations, necessitating comprehensive data analysis and trend evaluation for accurate revenue forecasting.
Customer Retention Rate
Customer Retention Rate, a vital business metric in data analysis, denotes the percentage of customers retained by a company over a given period. This metric highlights the customer satisfaction, loyalty, and engagement levels influenced by organizational strategies and service quality. Its unique feature lies in its ability to gauge customer relationship strength and brand loyalty, guiding businesses in developing personalized retention strategies and enhancing customer experience. While Customer Retention Rate serves as a valuable indicator of customer retention efforts, it may encounter limitations in capturing transient customer behaviors or external market influences, prompting businesses to integrate customer feedback and market analysis for comprehensive retention rate assessment.
Advanced KPI Examples
In the realm of data analysis, Advanced KPI Examples play a pivotal role. They serve as critical tools for assessing performance, predicting trends, and making informed decisions. By incorporating Advanced KPIs into analytical processes, data analysts can enhance the depth and accuracy of their insights. These examples go beyond basic metrics, delving into complex predictive analytics and real-time data assessment. Embracing Advanced KPIs signifies a dedication to precision and a forward-thinking approach in data analysis.
Predictive Analytics Metrics
Forecast Accuracy
Forecast Accuracy stands out as a paramount element within the spectrum of Advanced KPIs. It entails the ability to predict future trends and outcomes with precision based on historical data patterns. The key characteristic of Forecast Accuracy lies in its potential to minimize uncertainties and assist in strategic decision-making processes. Implementing Forecast Accuracy offers a strategic advantage by providing reasonable expectations and reducing the margin of error in forecasting trends. It equips data analysts with the means to anticipate changes and optimize performance effectively.
Customer Lifetime Value
Another crucial aspect of Advanced KPIs, Customer Lifetime Value, highlights the long-term worth of a customer to a business. Understanding Customer Lifetime Value empowers organizations to tailor their strategies to enhance customer relationships and maximize revenue streams. The key feature of Customer Lifetime Value lies in its ability to guide marketing strategies, retention efforts, and revenue forecasting. While advantageous in gauging customer loyalty and profitability, Customer Lifetime Value requires careful interpretation to avoid overlooking short-term gains and undervaluing immediate returns.
Machine Learning Evaluation Metrics
Model Accuracy
Among the Machine Learning Evaluation Metrics, Model Accuracy holds significant importance in validating the efficacy of predictive models. It denotes the degree of correctness in predictions made by machine learning algorithms. The key characteristic of Model Accuracy lies in its role in assessing the reliability and precision of predictive models. Opting for Model Accuracy ensures robust evaluation of machine learning algorithms, aiding in decision-making processes and model refinement. However, the reliance on Model Accuracy alone may overlook nuances that affect overall model performance, necessitating a comprehensive assessment approach.
Precision and Recall
Precision and Recall stand as fundamental components of Machine Learning Evaluation Metrics, emphasizing the balance between accuracy and completeness in model predictions. Precision denotes the proportion of relevant instances among the retrieved instances, while Recall measures the ability of the model to identify all relevant instances. The unique feature of Precision and Recall lies in their collaborative nature, offering insights into the model's ability to filter relevant information effectively. While beneficial in evaluating model performance comprehensively, Precision and Recall require nuanced interpretation to avoid skewing insights towards either precision or recall at the expense of overall model effectiveness.
Real-time Data KPIs
Data Latency
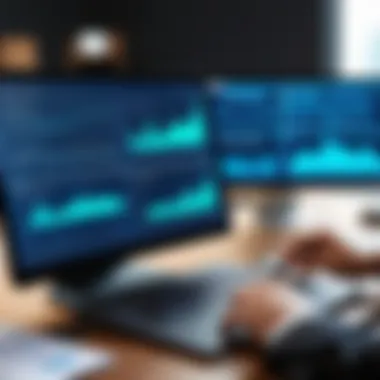
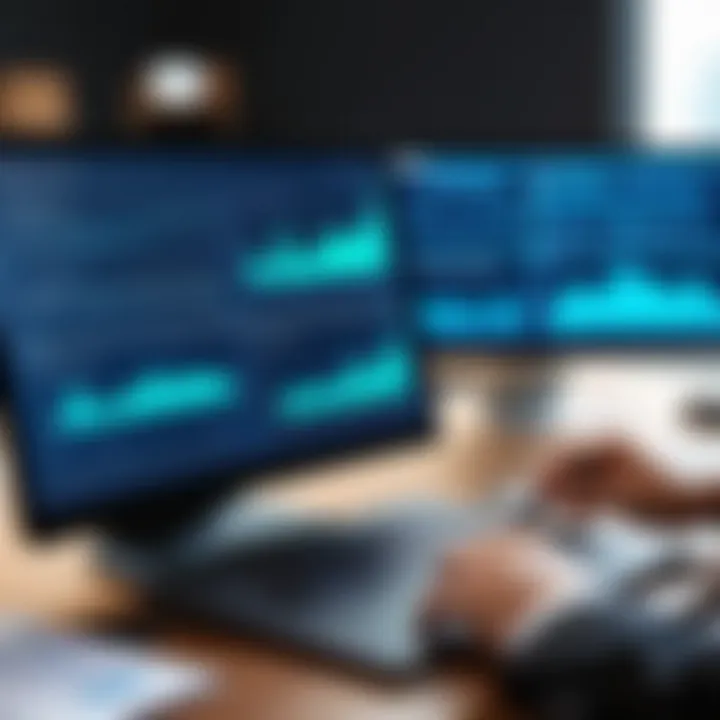
Within Real-time Data KPIs, Data Latency emerges as a critical facet, reflecting the time delay between data generation and data availability for analysis. The key characteristic of Data Latency lies in its impact on timely decision-making processes and the relevance of insights derived from real-time data. Prioritizing Data Latency enhances the responsiveness of analysis and ensures that decisions are based on up-to-date information. While advantageous in promoting dynamic decision-making, controlling Data Latency demands robust infrastructure and streamlined processes to mitigate delays and maintain data integrity.
Streaming Data Accuracy
Streaming Data Accuracy serves as a cornerstone of Real-time Data KPIs, emphasizing the precision and reliability of data processed in real-time streams. The key characteristic of Streaming Data Accuracy lies in its capacity to deliver immediate insights and support agile decision-making. Embracing Streaming Data Accuracy facilitates organizations in capturing instantaneous trends and responding promptly to emerging opportunities or challenges. However, maintaining high levels of Streaming Data Accuracy necessitates robust validation mechanisms and constant monitoring to uphold data integrity and analytical precision.
Implementing KPIs Effectively
In the landscape of data analysis, implementation of Key Performance Indicators (KPIs) stands paramount. It serves as the cornerstone for assessing the effectiveness of data-driven strategies and quantifying the progress towards predetermined objectives. Irrespective of the industry, implementing KPIs effectively revolves around strategic planning, meticulous execution, and continuous refinement to align analytical efforts with organizational goals. An integral aspect of this implementation is the seamless integration of KPIs across all levels of an organization, facilitating a cohesive approach towards data-driven decision-making. The benefits of effective KPI implementation span beyond performance evaluation, extending to fostering a data-centric culture, enhancing accountability, and streamlining operational processes for sustained growth and competitiveness within the dynamic data landscape.
Best Practices for KPI Implementation
Setting Clear Objectives
Setting clear objectives is a foundational pillar of effective KPI implementation. It entails defining specific, measurable, achievable, relevant, and time-bound (SMART) goals that align with the organization's strategic vision. Clear objectives serve as guiding beacons, enabling data analysts to focus their efforts on key metrics directly linked to business outcomes. By establishing clear objectives, organizations can enhance transparency, promote alignment across departments, and foster a shared understanding of performance expectations. The strategic deployment of clear objectives empowers data analysts to prioritize tasks efficiently, track progress accurately, and pivot strategies proactively based on real-time insights, driving tangible results and fostering a culture of continuous improvement.
Regular Monitoring and Evaluation
Regular monitoring and evaluation play a pivotal role in the success of KPI implementation initiatives. It involves setting up robust mechanisms to collect, analyze, and interpret data effectively to derive actionable insights. By integrating systematic monitoring and evaluation processes, organizations can gauge the performance of KPIs in real-time, identify deviations from predefined targets, and proactively address emerging challenges or opportunities. The cyclical nature of monitoring and evaluation fosters agility, enabling organizations to iterate strategies, recalibrate KPIs, and optimize decision-making processes based on data-driven feedback loops. Embracing regular monitoring and evaluation not only enhances the adaptive capacity of organizations but also cultivates a culture of learning, agility, and responsiveness to dynamic market conditions, ensuring sustained success in the realm of data analytics.
Ensuring Data Accuracy and Consistency
Data Validation Processes
Data validation processes are instrumental in safeguarding the integrity and reliability of data used to measure KPIs. These processes encompass a range of techniques and protocols aimed at verifying the quality, accuracy, and relevance of data inputs to prevent errors, inconsistencies, or biases that could distort analytical outcomes. By implementing rigorous data validation processes, organizations can instill confidence in decision-making processes, mitigate operational risks, and uphold the credibility of derived insights. Moreover, data validation enhances the robustness of predictive models, improves forecasting accuracy, and bolsters the efficacy of strategic initiatives by ensuring that data-driven decisions are anchored in trustworthy and verifiable information.
Error Handling Protocols
Error handling protocols play a critical role in fortifying the resilience of data analysis frameworks against unforeseen contingencies or anomalies. These protocols encompass predefined procedures, escalation pathways, and mitigation strategies designed to address errors, anomalies, or discrepancies that may arise during data collection, processing, or analysis. By instituting error handling protocols, organizations can streamline troubleshooting processes, expedite issue resolution, and maintain the integrity of analytical outputs even in the face of unexpected challenges. Effective error handling not only minimizes downtime and operational disruptions but also instills confidence in data quality, reinforces stakeholder trust, and ensures the consistent delivery of actionable insights essential for informed decision-making and sustainable business growth.
End
In the realm of data analysis, the Conclusion section serves as the compass that guides professionals towards the essence of their analytical endeavors. Delving deep into the intricacies of various Key Performance Indicators (KPIs), this article articulates a roadmap for data analysts to navigate the ocean of information intelligently. By dissecting the importance of KPIs in granular detail, this section sheds light on how these metrics act as beacons of performance measurement and decision-making in the data landscape. Ensuring a robust understanding of KPIs is pivotal for professionals striving for excellence in their analytical pursuits.
Key Takeaways
Crucial Role of KPIs in Data Analysis
Within the fabric of data analysis, the Crucial Role of KPIs emanates as a cornerstone of success. This pivotal aspect accentuates the essence of performance evaluation, offering a structured approach to gauge efficiency and effectiveness. The defining characteristic of this role lies in its ability to quantify abstract concepts into tangible metrics, enabling professionals to harness data insights strategically. Its inherent capacity to streamline decision-making processes catapults KPIs into the realm of indispensability, making them a fundamental choice for any data analyst looking to navigate the complexities of analytical landscapes. Despite its undeniable benefits, challenges may arise, such as the need for continuous calibration to ensure relevancy and accuracy.
Continuous Improvement through KPI Tracking
Embarking on the journey of Continuous Improvement through KPI Tracking paves the way for honing analytical skills and augmenting operational efficiencies. This facet of data analysis underscores a proactive approach towards enhancing performance by leveraging insights derived from KPI monitoring. The key characteristic of this practice lies in its iterative nature, fostering a culture of perpetual enhancement and refinement within analytical frameworks. Its value as a guiding compass for data professionals is underscored by its potential to unleash untapped opportunities for growth and innovation. While its advantages are manifold, vigilance is essential to navigate potential pitfalls, such as data overload or misinterpretation, thus emphasizing the importance of informed decision-making in KPI tracking initiatives.