Unveiling the Intricacies of Big Data: Significance, Applications, Challenges, and Future Trends
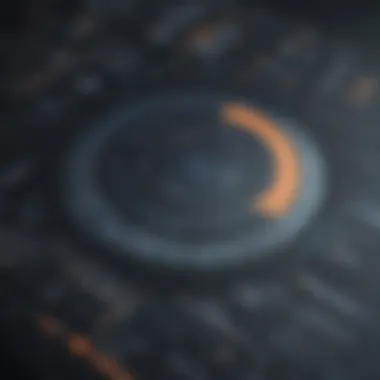
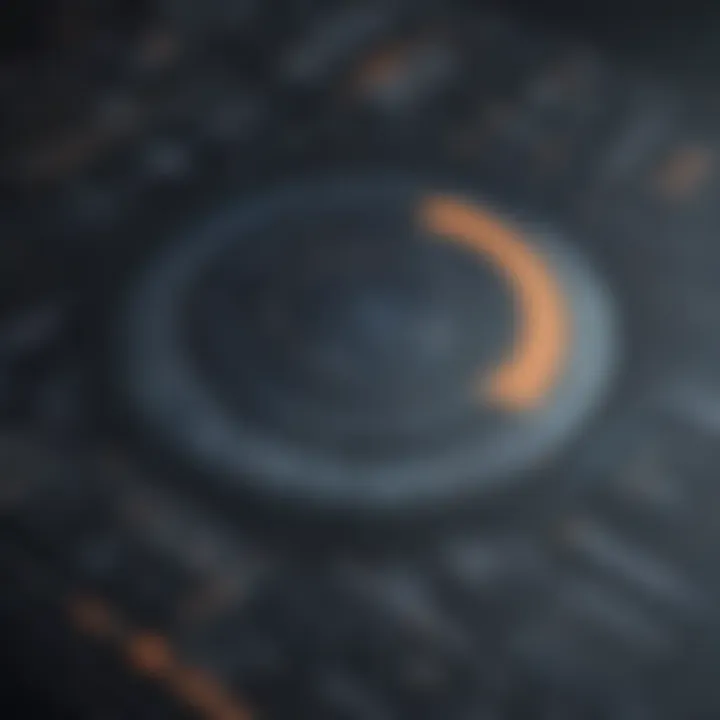
Overview of Topic
Big data is a revolutionary concept in the tech industry, transforming the way businesses operate and make decisions. The scope and significance of big data encompass vast amounts of information being analyzed to extract insights for strategic purposes. Understanding the evolution of big data provides a foundational context for its current applications and future advancements.
Fundamentals Explained
Core principles underlying big data involve the collection, storage, and analysis of massive datasets to reveal patterns, trends, and associations. Key terminology like data mining, machine learning, and data warehousing are essential to grasp the functional aspects of big data. Basic concepts such as structured and unstructured data, predictive analytics, and data visualization form the groundwork for delving deeper into big data.
Practical Applications and Examples
Real-world applications of big data range from customer analytics in e-commerce to predictive maintenance in manufacturing. Case studies showcasing how big data has revolutionized healthcare, finance, and marketing provide tangible examples of its impact. Hands-on projects involving data processing, modeling, and interpretation equip learners with practical insights into implementing big data solutions.
Advanced Topics and Latest Trends
Embracing cutting-edge developments in big data involves exploring techniques like deep learning, natural language processing, and edge analytics. Advanced methodologies such as neural networks and ensemble modeling push the boundaries of data analysis. Identifying future trends like edge computing, big data ethics, and quantum computing paves the way for anticipating innovations in the field.
Tips and Resources for Further Learning
Recommended resources for individuals seeking to enhance their understanding of big data include books like 'Big Data Revolution' by Rob Thomas and Billy Bosworth. Online courses from platforms like Coursera and Udemy offer specialized modules on big data technologies. Tools and software like Hadoop, Tableau, and Spark provide practical avenues for applying big data concepts in real-world scenarios.
Introduction to Big Data
Big Data, a pivotal element in the digital landscape, holds immense significance in modern technology. This section delves into the fundamental concepts underpinning the vast realms of data analysis and interpretation. Introduction to Big Data acts as the gateway to a profound understanding of information processing, offering a bird's eye view of the transformative power embedded within colossal datasets. Readers are encouraged to explore the intricate nuances and intricate systems powering the Big Data domain.
Defining Big Data
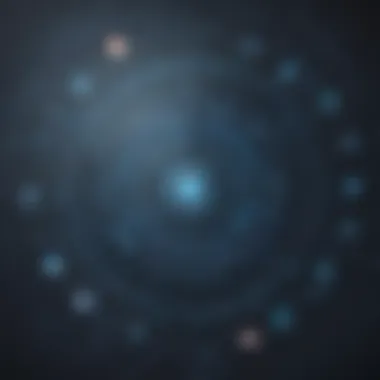
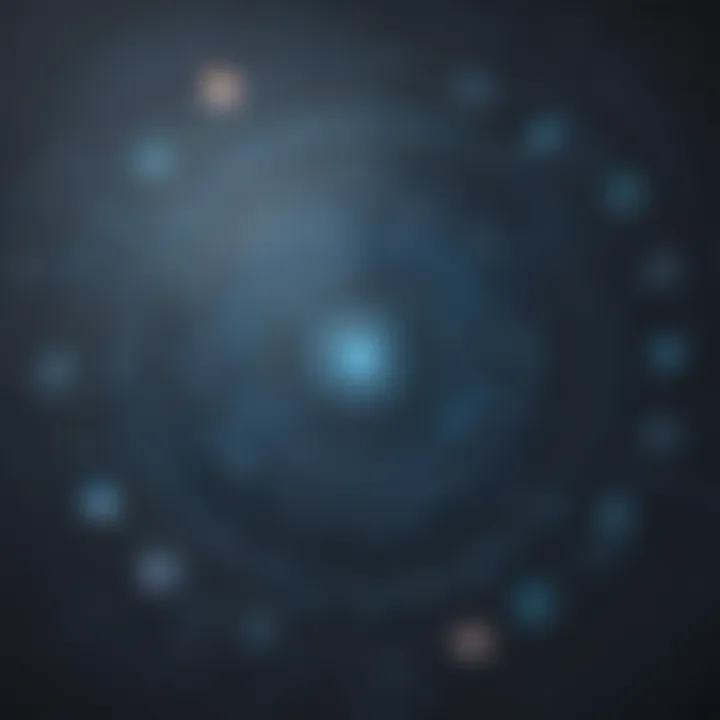
Volume
Volume, a core component of Big Data, denotes the sheer quantity of data generated and collected across various sources. The exponential growth of data volume in recent years necessitates robust mechanisms for storage and analysis. Its key characteristic lies in the vastness and diversity of information accessible for analysis. Volume, owing to its magnitude, is a quintessential aspect discussed in this article, shedding light on its implications and strategic advantages.
Velocity
Velocity refers to the speed at which data is created and processed, a crucial aspect in the Big Data ecosystem. Rapid data generation necessitates real-time processing capabilities to extract actionable insights. This article elucidates the significance of velocity in data analytics, emphasizing its role in driving dynamic decision-making processes.
Variety
Variety encompasses the diverse forms of data types and sources available for analysis within the Big Data landscape. From structured to unstructured data, the variety presents both challenges and opportunities for data scientists and analysts. This section examines the multifaceted nature of data variety, highlighting its impact on information interpretation and utilization.
Veracity
Veracity focuses on the accuracy and reliability of data sources, influencing the trustworthiness of insights derived from Big Data analytics. Ensuring data veracity is essential for making informed decisions based on credible information. This article explores the intricacies of data veracity, underscoring its vital role in mitigating risks and enhancing the quality of analytical outcomes.
Importance of Big Data
Business Insights
Business Insights derived from Big Data analytics offer organizations valuable perspectives on market trends, consumer behavior, and operational efficiencies. By harnessing the power of data analytics, companies can make informed decisions, optimize processes, and gain a competitive edge in the market. This section elaborates on the pivotal role of business insights in leveraging Big Data for strategic growth and development.
Decision Making
Effective Decision Making is facilitated by the insights gleaned from Big Data analysis, empowering leadership to respond promptly to changing market dynamics and consumer demands. The ability to extrapolate actionable intelligence from vast datasets enhances the decision-making process, leading to more informed and impactful strategies. This segment delves into the nuances of decision-making within the realm of Big Data, emphasizing its transformative potential.
Predictive Analytics
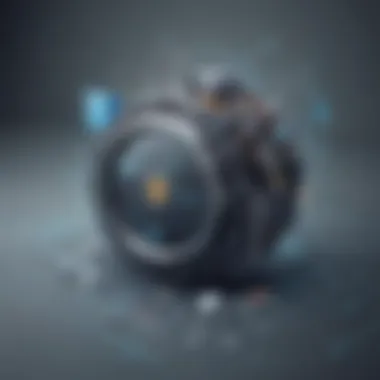
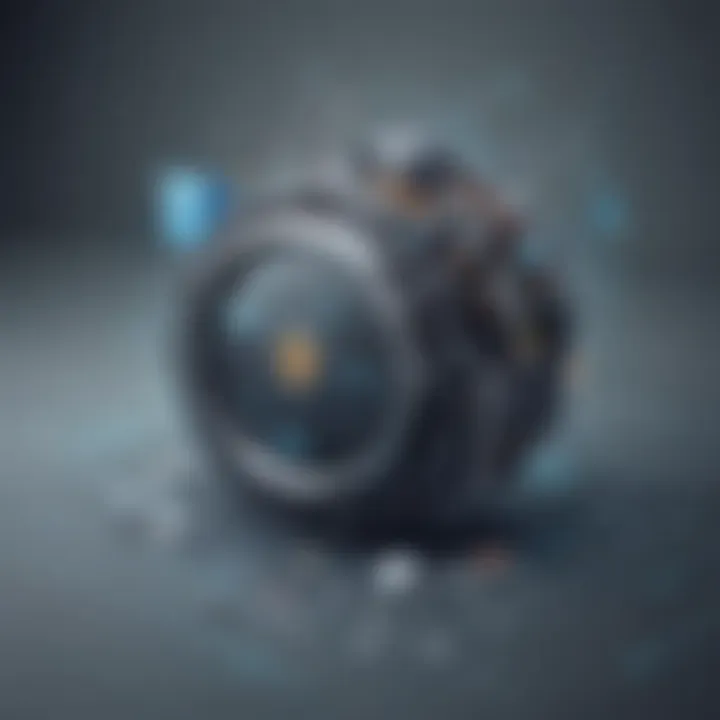
Predictive Analytics leverages historical data trends and statistical models to forecast future outcomes and trends. This proactive approach enables organizations to anticipate market shifts, customer preferences, and potential risks, optimizing resource allocation and strategic planning. The discussion in this article elucidates the power of predictive analytics in driving growth and innovation across various industry sectors.
Applications of Big Data
Finance
In the financial sector, Big Data analytics revolutionizes risk management, fraud detection, and algorithmic trading. The analysis of vast financial datasets enables institutions to identify patterns, mitigate risks, and optimize investment portfolios. This section explores the transformative impact of Big Data in enhancing financial operations and decision-making processes.
Healthcare
Big Data applications in healthcare encompass predictive diagnostics, personalized medicine, and population health management. By analyzing patient data and clinical trends, healthcare providers can deliver tailored treatments, improve outcomes, and optimize care delivery. This article delves into the significant role of Big Data in transforming the healthcare industry and improving patient-centric services.
Marketing
In the marketing domain, Big Data unlocks valuable insights into consumer behavior, market trends, and campaign performance. By analyzing customer demographics and engagement metrics, marketers can refine targeting strategies, personalize messaging, and increase ROI. This segment discusses the disruptive impact of Big Data on marketing practices and customer engagement.
Transportation
The transportation sector leverages Big Data for route optimization, predictive maintenance, and traffic management. By analyzing real-time traffic data and logistical patterns, transport companies can enhance operational efficiency, reduce costs, and improve customer experience. This section delves into the innovative applications of Big Data in revolutionizing transportation services and infrastructure.
Telecommunications
In the telecommunications industry, Big Data analytics supports network optimization, customer segmentation, and predictive maintenance. By analyzing user behavior and network performance data, telecom operators can enhance service quality, prevent outages, and drive customer satisfaction. This article elucidates the critical role of Big Data in transforming telecommunications operations and driving technological advancements.
Challenges in Handling Big Data

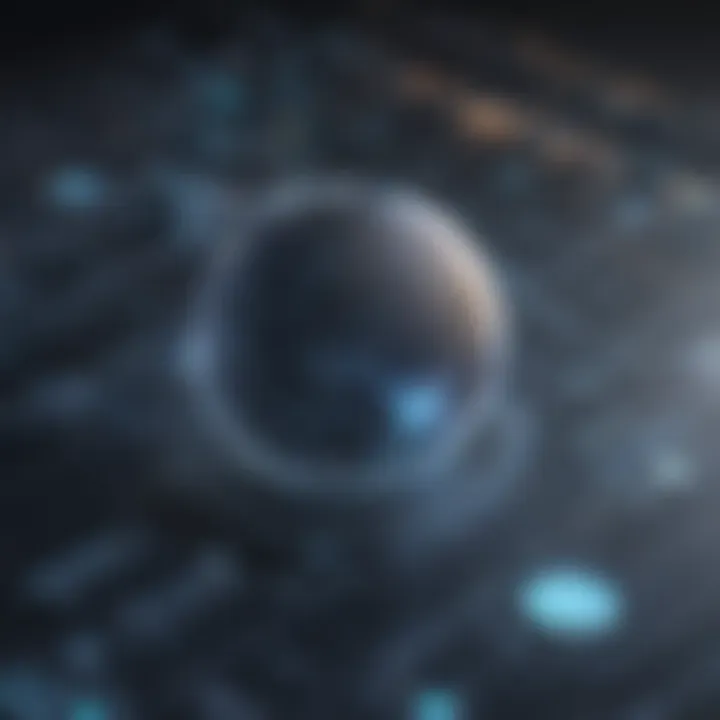
Data Security
Data Security concerns the protection of sensitive information from unauthorized access, breaches, or cyber threats. Safeguarding data integrity and confidentiality is paramount in the Big Data ecosystem to prevent data breaches and compliance violations. This section delves into the complexities of data security in the context of Big Data analytics, highlighting the importance of robust security measures and protocols.
Data Privacy
Data Privacy focuses on the ethical and legal considerations surrounding the collection, storage, and usage of personal data. Ensuring data privacy compliance and respecting user rights are essential aspects of responsible data handling practices. This article addresses the challenges of data privacy in the era of Big Data, emphasizing the need for transparency and accountability in data processing.
Data Quality
Data Quality pertains to the accuracy, consistency, and reliability of data sources used for analysis and decision-making. Poor data quality can lead to erroneous insights, flawed strategies, and business inefficiencies. This segment sheds light on the significance of data quality in the Big Data environment, emphasizing quality assurance measures and best practices for ensuring data accuracy and integrity.
Scalability
Scalability refers to the capacity of systems and infrastructure to accommodate growing data volumes and processing demands. The ability to scale resources dynamically is crucial for handling large-scale data operations efficiently. This section explores the challenges and strategies associated with scalability in Big Data analytics, emphasizing the need for flexible and resilient infrastructure solutions.
Future Trends in Big Data
Machine Learning Integration
Machine Learning Integration enhances the predictive capabilities of Big Data analytics by enabling algorithms to learn from data patterns and improve predictions over time. The integration of machine learning algorithms empowers data scientists to uncover complex relationships, automate decision-making processes, and drive innovation. This segment delves into the evolving landscape of machine learning integration in Big Data analytics, highlighting its transformative potential and future applications.
Edge Computing
Edge Computing facilitates real-time data processing and analysis at the edge of the network, minimizing latency and bandwidth usage. By distributing computation closer to data sources, edge computing accelerates response times and enhances operational efficiency. This article discusses the growing prominence of edge computing in enabling agile and scalable data analytics solutions within the Big Data domain.
Ethical Data Handling
Ethical Data Handling emphasizes the responsible and transparent use of data assets, considering privacy, consent, and fairness in data practices. Upholding ethical standards in data collection, storage, and analysis is imperative to build trust with stakeholders and regulatory compliance. This section addresses the emerging importance of ethical data handling in the age of Big Data, emphasizing the ethical considerations and guidelines for data governance.
Enhanced Data Visualization
Enhanced Data Visualization leverages advanced tools and techniques to represent complex data sets in visually compelling formats. By harnessing interactive visuals and intuitive interfaces, data visualization enhances data interpretation, decision-making, and communication of insights. This segment explores the role of enhanced data visualization in making data more accessible, meaningful, and actionable in the Big Data landscape.