Unleashing Data Analyst Excellence: Mastering Performance Metrics
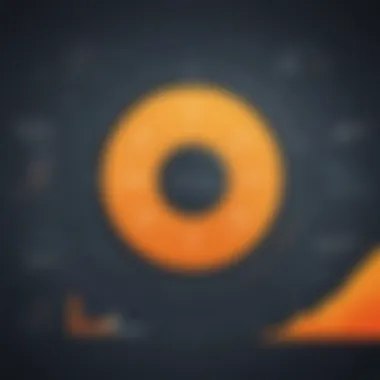
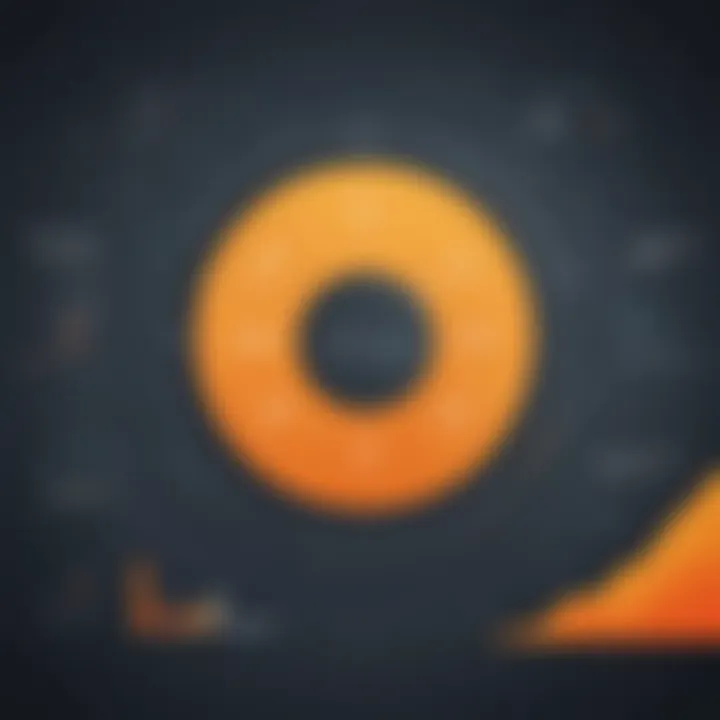
Overview of Topic
Fundamentals Explained
Practical Applications and Examples
Real-world case studies and practical applications serve as invaluable learning tools for aspiring data analysts. By exploring demonstrations and hands-on projects, individuals can apply theoretical knowledge to solve actual data-related problems. Implementing code snippets and following specific guidelines further enhance the practicality of these examples, allowing for a deeper understanding of how optimizing performance metrics can directly impact data analysis outcomes.
Advanced Topics and Latest Trends
In the ever-evolving field of data analytics, staying updated on cutting-edge developments is imperative for data analysts seeking to excel in their roles. Advanced techniques such as machine learning algorithms, predictive modeling, and artificial intelligence are revolutionizing data analysis methodologies. Examining future prospects and upcoming trends enables data analysts to anticipate industry shifts and proactively adapt to new technologies for enhanced performance.
Tips and Resources for Further Learning
Introduction
In the realm of data analysis, the maximization of performance metrics holds paramount significance. Understanding and utilizing these metrics efficiently can significantly enhance the productivity and output of data analysts. By delving deep into key indicators, this article aims to provide a detailed analysis of how these metrics play a crucial role in evaluating and maximizing the efficiency and effectiveness of data analysts.
Understanding Data Analyst Performance Metrics
Defining the role of data analysts
Defining the role of data analysts is pivotal in grasping the core responsibilities and functions they undertake within an organization. This includes tasks such as data collection, analysis, interpretation, and presentation. Such a definition serves as the foundation for establishing performance metrics that align with the objectives of data analysts. The uniqueness of defining the role lies in its ability to act as a roadmap for setting expectations and evaluating the performance of data analysts based on predefined criteria. This structured approach allows for a systematic evaluation of data analyst contributions, thereby streamlining performance management processes.
Importance of performance metrics in data analysis
The importance of performance metrics in data analysis cannot be overstated. These metrics serve as quantifiable measures that assess the effectiveness and efficiency of data analysts in their roles. By highlighting areas of strength and areas needing improvement, performance metrics enable organizations to enhance their overall data analysis capabilities. The key characteristic of using performance metrics lies in its ability to provide a standardized framework for evaluating and benchmarking data analyst performance consistently. This approach aids in identifying patterns, trends, and outliers, offering valuable insights for optimizing performance and making informed decisions. Despite its numerous benefits, implementing performance metrics may pose challenges related to data quality, metric relevancy, and interpretational complexities, which organizations need to address to leverage the full potential of such metrics.
Key Performance Metrics for Data Analysts
In the realm of data analysis, Key Performance Metrics play a pivotal role in evaluating and enhancing the performance of data analysts. These metrics serve as crucial indicators of productivity, accuracy, efficiency, and innovation within the data analysis process. By carefully monitoring and optimizing these metrics, organizations can drive improvements in data analyst performance, leading to enhanced decision-making and business outcomes.
Productivity Metrics
Productivity Metrics are fundamental in assessing the output and efficiency of data analysts. One key aspect is the 'Number of analyses conducted.' This metric quantifies the total volume of analyses completed within a specific time frame, providing insights into the workload and output of data analysts. It helps measure the efficiency of data analysts in handling tasks and delivering results promptly. Another critical metric is the 'Average time taken per analysis,' which highlights the speed and effectiveness of data analysts in completing individual tasks. By evaluating this metric, organizations can identify bottlenecks in the analysis process and optimize workflows for enhanced productivity.
Number of analyses conducted
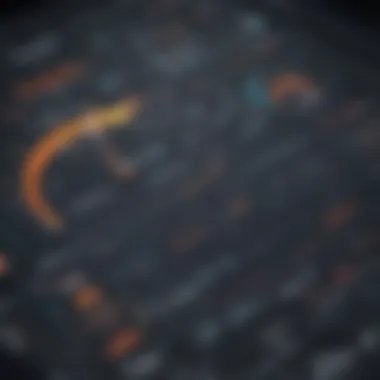
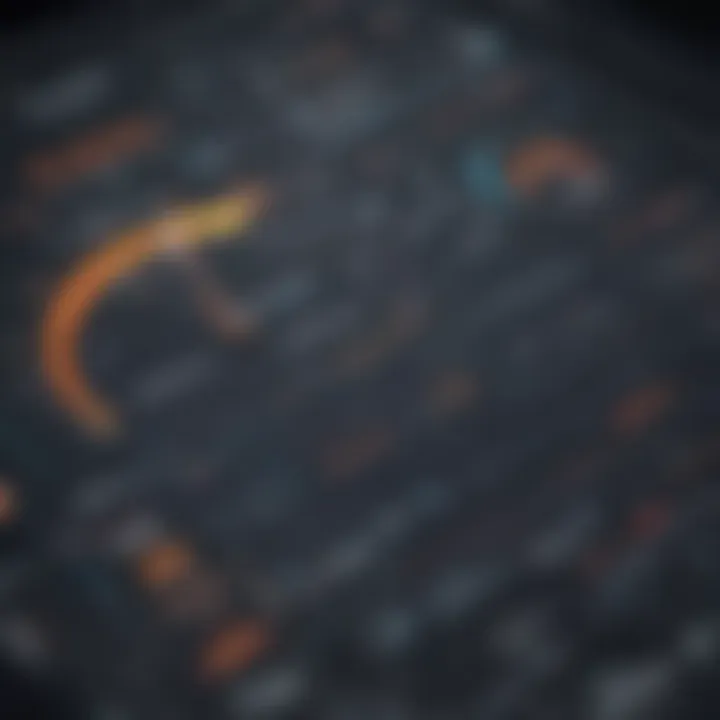
The 'Number of analyses conducted' metric is a vital measure that showcases the workload and productivity of data analysts. By tracking this metric, organizations can gain visibility into the capacity and performance of their data analysts. A higher number of analyses conducted indicates increased productivity and efficiency, reflecting positively on the overall operational efficiency of the data analysis team. However, it is essential to balance quantity with quality to ensure that the analyses performed are thorough and accurate.
Average time taken per analysis
The 'Average time taken per analysis' metric sheds light on the efficiency and effectiveness of data analysts in completing individual tasks. A shorter average time implies faster turnaround and better resource utilization, contributing to enhanced productivity. However, excessively low times may indicate rushed or incomplete analyses, compromising accuracy. By carefully monitoring and optimizing this metric, organizations can strike a balance between speed and thoroughness, optimizing the overall performance of data analysts.
Accuracy Metrics
Accuracy Metrics are paramount in ensuring the quality and reliability of data analysis processes. 'Error rates in analysis' is a critical aspect that measures the frequency and severity of inaccuracies in analytical outputs. By identifying and mitigating errors, organizations can enhance the trustworthiness of analytical results and avoid costly mistakes. Additionally, 'Data quality assessments' evaluate the overall integrity and consistency of data used in analyses, safeguarding against inaccuracies and bias in decision-making.
Error rates in analysis
The 'Error rates in analysis' metric is essential in quantifying the accuracy and reliability of analytical outputs. High error rates indicate potential issues in data processing, methodology, or interpretation, warranting immediate attention to rectify errors and improve accuracy. By investigating the root causes of errors and implementing corrective measures, organizations can elevate the quality and precision of their analytical outcomes.
Data quality assessments
'Data quality assessments' focus on evaluating the completeness, accuracy, and reliability of data sources employed in analyses. By conducting rigorous assessments, organizations can ensure that the data utilized is free from errors, inconsistencies, and biases, resulting in more robust and trustworthy analytical insights. Maintaining high standards of data quality is essential for informed decision-making and reliable business intelligence.
Efficiency Metrics
Efficiency Metrics are instrumental in optimizing the operational workflows and resource utilization of data analysts. 'Time spent on data preparation' measures the duration allocated to data cleaning, preprocessing, and transformation tasks, impacting the overall efficiency and timeliness of analyses. Furthermore, 'Utilization of analytical tools' evaluates the effectiveness and proficiency of data analysts in leveraging analytical software and technologies to streamline processes and enhance analytical capabilities.
Time spent on data preparation
'Time spent on data preparation' quantifies the investment of resources in priming and refining data for analysis. Minimizing the time spent on preparatory tasks enhances the agility and responsiveness of data analysts, allowing them to focus on deriving insights and value from data. Efficient data preparation processes support faster decision-making and enable organizations to extract meaningful information promptly from diverse datasets.
Utilization of analytical tools
'Utilization of analytical tools' assesses the adeptness and proficiency of data analysts in leveraging analytical software and tools to expedite data analysis tasks. Proficient utilization of tools enhances the scalability and capacity of data analysts to handle complex datasets and perform advanced analyses. By optimizing tool usage and exploring advanced functionalities, organizations can maximize the efficiency and effectiveness of their data analysis endeavors.
Innovation Metrics
Innovation Metrics spotlight the creative and forward-thinking capabilities of data analysts in exploring new techniques and generating impactful visualizations. 'Introduction of new techniques' signifies the adoption of novel methodologies and approaches to data analysis, fostering innovation and continuous improvement in analytical practices. Moreover, 'Creation of impactful visualizations' underscores the importance of presenting data in a compelling and insightful manner, enabling stakeholders to grasp complex information quickly and make data-driven decisions.
Introduction of new techniques
The 'Introduction of new techniques' metric highlights the proactive pursuit of innovative methods and tools in data analysis. By embracing new techniques, data analysts can enhance the depth and breadth of their analytical capabilities, uncovering insights and patterns previously overlooked. Experimenting with cutting-edge approaches empowers organizations to stay ahead of trends and harness the full potential of their data assets for strategic decision-making.
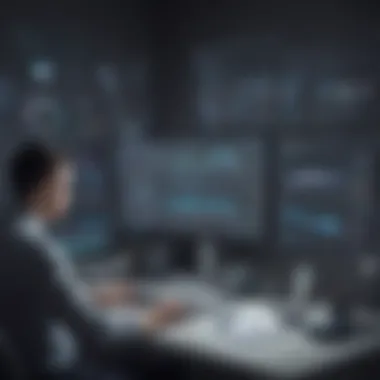
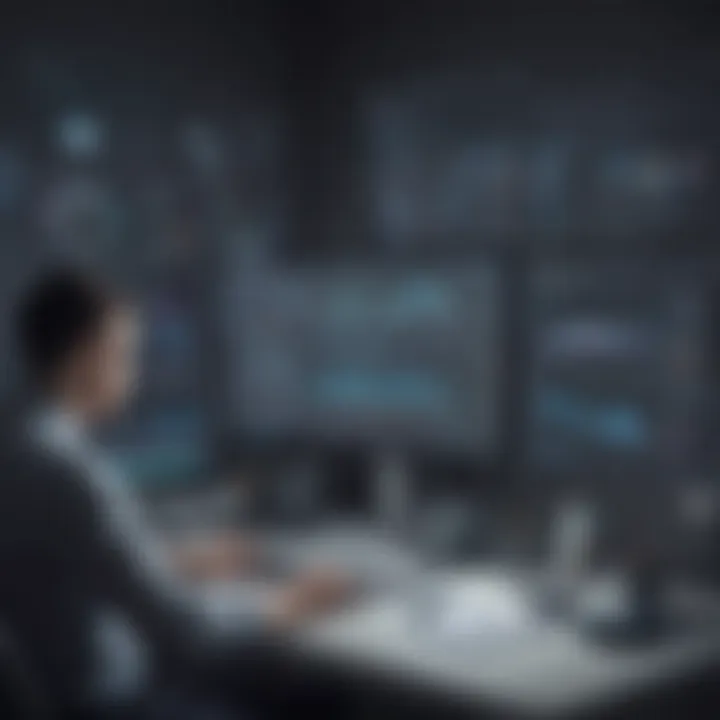
Creation of impactful visualizations
'Creation of impactful visualizations' focuses on the art of transforming data into visually engaging and informative representations. Effective visualization techniques amplify the comprehension and communication of complex data insights, enabling stakeholders to grasp information quickly and discern key trends effortlessly. By investing in impactful visualization practices, organizations can enhance the interpretability and relevance of analytical findings, driving informed decision-making and strategic actions.
Implementing Effective Metrics Tracking
Choosing the Right Metrics
Aligning metrics with business objectives
Aligning metrics with business objectives is a critical aspect within the realm of effective metrics tracking. It ensures that the chosen metrics directly contribute to achieving the organization's overarching goals. By aligning metrics with business objectives, companies can strategically measure the performance of data analysts in a manner that reflects the desired outcomes of their data analysis efforts. This alignment allows for a more purposeful evaluation of analysts' contributions towards organizational success, thereby enhancing decision-making processes and resource allocations based on tangible results. However, a potential challenge of aligning metrics with business objectives lies in the complexity of mapping abstract strategic goals to specific quantifiable metrics accurately. Yet, the benefits of this alignment far outweigh the challenges, as it provides a clear roadmap for data analyst evaluations that resonate with the larger organizational mission.
Balancing quantitative and qualitative indicators
Balancing quantitative and qualitative indicators is a vital consideration when selecting the right metrics for evaluating data analyst performance. While quantitative metrics offer tangible measurements such as the number of analyses conducted and time taken per analysis, qualitative indicators dive deeper into areas like data accuracy assessments and innovative visualization creations. Achieving a balance between these two types of metrics ensures a holistic evaluation of data analyst capabilities, where both the output volumes and the quality of the analyses are taken into account. This balance is essential for providing a comprehensive understanding of the analysts' effectiveness, allowing organizations to make informed decisions that consider both the quantity and quality dimensions of their data analysis efforts.
Establishing Data Collection Procedures
Utilizing automation tools
The integration of automation tools within data collection procedures plays a pivotal role in ensuring efficiency and accuracy in metrics tracking. By utilizing automation tools, organizations can streamline the data collection process, reducing manual intervention and the likelihood of errors. Automation tools enable the seamless extraction of relevant performance data, ensuring timely and consistent metric updates for thorough analysis and evaluation. The key characteristic of utilizing automation tools lies in its ability to enhance the speed and accuracy of data collection, providing real-time insights into data analyst performance metrics. However, organizations should be mindful of potential drawbacks, such as the initial setup costs and the need for regular maintenance to ensure the proper functioning of these tools.
Ensuring data accuracy and consistency
Ensuring data accuracy and consistency is a foundational element of establishing robust data collection procedures. By maintaining data accuracy and consistency, organizations can rely on the integrity of the performance metrics tracked to make informed decisions. Data accuracy pertains to the precision and correctness of the collected metrics, while data consistency ensures uniformity and reliability in the measurement process over time. The unique feature of ensuring data accuracy and consistency lies in its role as a fundamental pillar for effective metrics tracking, forming the basis for meaningful analysis and interpretation. While the benefits of maintaining data accuracy and consistency are significant, organizations must allocate resources to periodically validate and verify the collected data to mitigate the risk of inaccuracies or discrepancies.
Analyzing and Interpreting Metrics Data
Identifying trends and patterns
Identifying trends and patterns within metrics data is instrumental in extracting actionable insights for enhancing data analyst performance. By recognizing recurring trends and patterns, organizations can identify areas of strength and areas that require improvement among data analysts. This process enables organizations to capitalize on successful strategies and address underlying issues promptly, fostering continuous performance enhancement. The key characteristic of identifying trends and patterns is its ability to uncover hidden correlations and dependencies within the metrics data that may not be immediately apparent. This analytical approach empowers organizations to make data-informed decisions that drive positive outcomes and optimize data analyst productivity effectively.
Deriving actionable insights for improvement
Deriving actionable insights for improvement from metrics data is paramount for maximizing data analyst performance. By extracting actionable insights, organizations can proactively address performance gaps, implement targeted interventions, and enhance overall efficiency and effectiveness. The unique feature of deriving actionable insights for improvement lies in its applicability for initiating meaningful changes based on data-driven findings. These insights offer concrete recommendations for optimizing data analyst performance, facilitating continuous growth and development within the organization. However, organizations must also consider the potential challenges of interpreting complex metrics data and transforming insights into actionable strategies to realize tangible improvements in data analyst performance.
Optimizing Data Analyst Performance
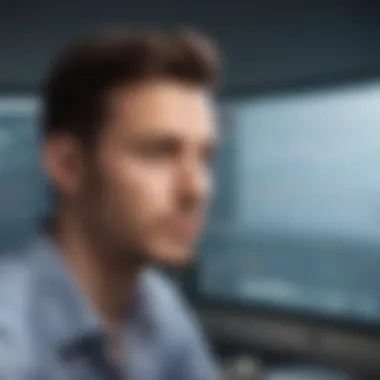
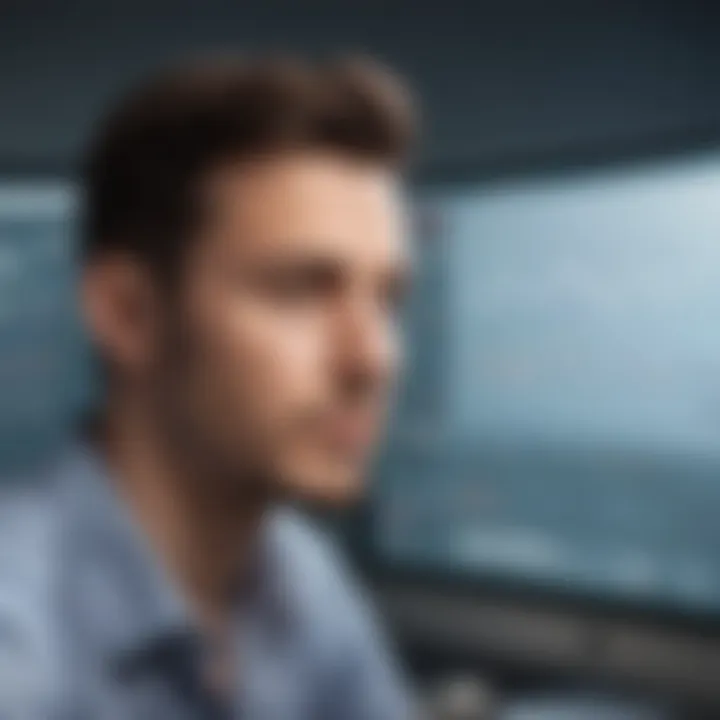
In the realm of data analysis, optimizing data analyst performance holds paramount importance. To ensure maximum efficiency and effectiveness in data analysis processes, it is crucial to focus on enhancing data analyst performance metrics. By continuously refining and optimizing performance, data analysts can significantly contribute to organizational success. Through a strategic approach to performance optimization, data analysts can streamline workflows, improve decision-making processes, and enhance overall data analysis outcomes.
Continuous Learning and Development
Encouraging skills enhancement
Encouraging skills enhancement plays a pivotal role in the overall goal of optimizing data analyst performance. By promoting continuous learning and skill development among data analysts, organizations can cultivate a culture of innovation and expertise. This aspect emphasizes the importance of staying updated with the latest technologies, tools, and techniques relevant to the field of data analysis. Encouraging skills enhancement not only enhances individual proficiency but also contributes to the collective knowledge base of the team, fostering a collaborative and dynamic work environment.
Promoting ongoing training programs
Promoting ongoing training programs is instrumental in maximizing data analyst performance. By offering structured training sessions, workshops, and courses, organizations can empower data analysts to acquire new skills and deepen their understanding of complex analytical concepts. Ongoing training programs help bridge skill gaps, nurture talent, and promote continuous improvement. With access to continuous learning opportunities, data analysts can stay abreast of industry trends, best practices, and emerging technologies, equipping them to tackle diverse analytical challenges with confidence.
Collaboration and Communication
Building cross-functional partnerships
Building cross-functional partnerships enriches the collaborative ecosystem within data analysis teams, fostering synergy and knowledge exchange. By forging connections with professionals from different domains such as marketing, finance, and operations, data analysts can gain valuable insights and diverse perspectives. Collaborating with cross-functional teams not only enhances the quality of analysis but also encourages interdisciplinary collaboration, resulting in more robust and innovative solutions.
Enhancing reporting mechanisms
Enhancing reporting mechanisms streamlines communication channels and ensures the efficient dissemination of analytical findings. By developing clear and actionable reports, data analysts can effectively communicate insights, trends, and recommendations to key stakeholders. Improved reporting mechanisms enable decision-makers to make informed choices based on data-driven evidence, promoting transparency and accountability within the organization.
Feedback and Performance Reviews
Regular performance evaluations
Regular performance evaluations provide a structured framework for assessing data analyst performance against predetermined metrics and objectives. By conducting periodic reviews, organizations can identify strengths, areas for improvement, and development opportunities for data analysts. Regular performance evaluations not only facilitate performance tracking but also serve as a platform for setting performance goals, recognizing achievements, and offering constructive feedback for continuous growth.
Constructive feedback mechanisms
Constructive feedback mechanisms play a pivotal role in nurturing a culture of continuous improvement and learning within data analysis teams. By providing constructive feedback, managers and peers can offer targeted suggestions for enhancing performance, refining analytical approaches, and addressing skill gaps. Constructive feedback mechanisms promote open communication, empower data analysts to thrive in their roles, and foster a culture of excellence and accountability.
Conclusion
In the realm of data analyst performance metrics, the Conclusion section plays a pivotal role by wrapping up the key insights discussed throughout the article. It serves as a crucial roadmap for readers, guiding them towards implementing effective strategies to maximize data analyst performance metrics. By summarizing the significance of leveraging performance metrics and emphasizing the holistic approach required for evaluating data analysts, this section encapsulates the essence of ensuring efficiency and efficacy in data analysis processes.
Summarizing Data Analyst Performance Metrics
Recap of Key Metrics Discussed
When delving into the Recap of key metrics discussed, the focus lies on condensing the essential indicators highlighted earlier in the article. This detailed review serves as a reference point for gauging data analyst performance and productivity. The Recap section aims to illuminate the measurable aspects crucial for evaluating the effectiveness of data analysts. By dissecting key metrics such as productivity, accuracy, efficiency, and innovation, readers gain insights into optimizing the output of their data analysts effectively. The Recap not only acts as a compass for data analysis practices but also underscores the significance of tracking and utilizing these metrics to enhance operational excellence within organizations.
Importance of a Holistic Approach to Performance Evaluation
The Importance of a holistic approach to performance evaluation underscores the necessity of considering both quantitative and qualitative metrics when assessing data analyst performance. By embracing a comprehensive evaluation method that transcends mere numerical values, organizations can cultivate a deeper understanding of the overall impact of their data analysts. This approach fosters a culture of continuous improvement by enabling stakeholders to garner insights beyond surface-level statistics. The holistic evaluation framework emphasizes the multifaceted nature of data analytics, highlighting the interplay between various metrics and their combined influence on organizational outcomes. By adopting this integrated approach, businesses can elevate their analytical capabilities and drive data-driven decision-making processes with more profound clarity and precision.