Unlocking the Profound Depths of Data Analysis: A Comprehensive Exploration
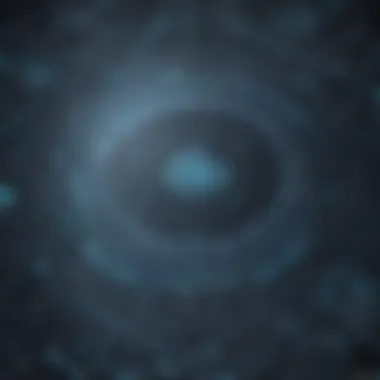
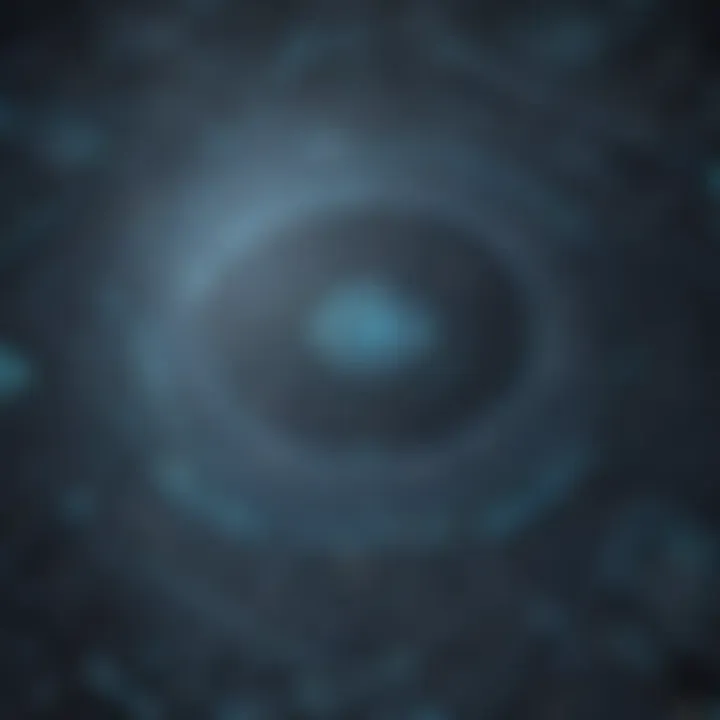
Overview of Unlocking the Depths of Data Analysis
In this section, we will delve into the intricate world of data analysis in research, exploring its meaning, methods, and significance. Data analysis holds a vital role in today's tech industry, driving crucial decision-making processes across various sectors. Understanding the fundamentals of data analysis is imperative for individuals aiming to excel in fields like data science, AI, and machine learning. This section will provide a brief historical background, tracing the evolution of data analysis from its nascent stages to its current pivotal position in driving innovation and progress.
Fundamentals of Data Analysis Unveiled
As we venture deeper into the complexities of data analysis, it becomes essential to grasp the core principles and theories underpinning this field. Key terminologies and definitions play a pivotal role in shaping our comprehension of data analysis methodologies. Exploring basic concepts and foundational knowledge equips individuals with the necessary framework to navigate the intricate landscape of data analysis with proficiency and insight.
Bringing Data Analysis to Life: Practical Applications and Examples
To cement our understanding of data analysis, real-world case studies and practical applications serve as invaluable tools. By dissecting demonstrations and engaging in hands-on projects, readers can witness firsthand the transformative power of data analysis in diverse scenarios. Code snippets and implementation guidelines offer a hands-on approach, enabling individuals to bridge the gap between theory and practical application, fostering a holistic understanding of data analysis techniques.
Embracing the Future: Advanced Topics and Latest Trends in Data Analysis
The field of data analysis is constantly evolving, with cutting-edge developments redefining traditional approaches. Exploring advanced techniques and methodologies enriches our understanding of data analysis, paving the way for innovative solutions and groundbreaking discoveries. By delving into future prospects and upcoming trends, readers can chart a course towards staying abreast of the dynamic landscape of data analysis, positioning themselves as trailblazers in the field.
Guidance for Continuous Learning: Tips and Resources
For individuals seeking to delve deeper into the realms of data analysis, a curated list of recommended books, courses, and online resources awaits. Additionally, tools and software geared towards practical usage empower readers to translate theoretical knowledge into actionable insights. By leveraging these resources, individuals can embark on a continuous learning journey, honing their data analysis skills and propelling their career trajectory into realms of unprecedented growth and innovation.
Introduction to Data Analysis in Research
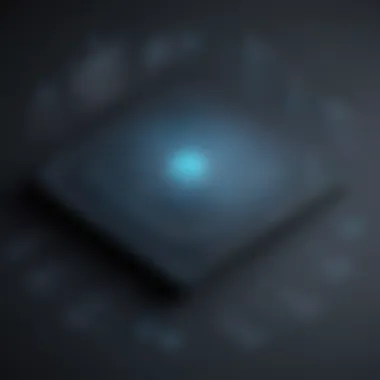
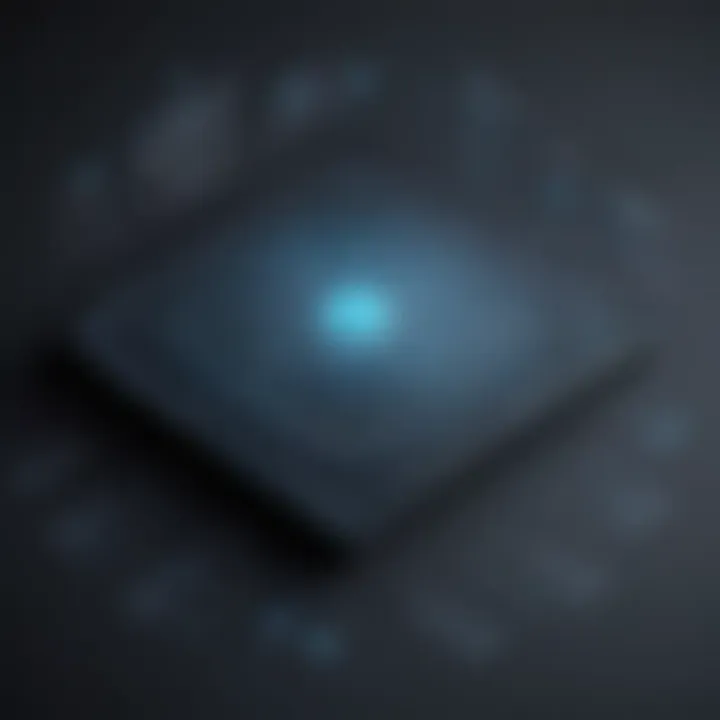
Data analysis in research plays a pivotal role in deriving valuable insights from data. It serves as the foundation for understanding trends, patterns, and correlations within datasets. In this article, we will delve into the intricacies of data analysis, highlighting its significance and application in research settings. By examining the various methodologies and techniques of data analysis, readers will gain a profound understanding of its role in facilitating evidence-based decision-making processes.
Defining Data Analysis
Understanding the essence of data analysis
Understanding the essence of data analysis involves grasping the fundamental principles that underpin this process. It focuses on dissecting raw data to extract meaningful information essential for research and decision-making. The key characteristic of understanding the essence of data analysis lies in its ability to transform complex datasets into actionable insights. By emphasizing data interpretation and visualization, this aspect enhances the comprehension of intricate data structures.
Importance of data analysis in research
The importance of data analysis in research cannot be overstated, as it serves as the backbone of empirical investigations. Its contribution lies in validating research hypotheses, uncovering hidden patterns, and refining theories based on empirical evidence. The key characteristic of the importance of data analysis is its capacity to enhance research validity by ensuring data-driven conclusions. While its advantages include efficiency and reliability, potential disadvantages may arise from misinterpretation of findings or data biases.
Scope of Data Analysis
The breadth of data analysis methodologies
The breadth of data analysis methodologies encompasses a wide array of techniques and tools employed to analyze diverse datasets. It allows researchers to choose suitable approaches based on the nature of data and research objectives. The key characteristic of the breadth of data analysis methodologies is its adaptability and versatility in handling different types of data. Its unique feature lies in the integration of statistical models with practical applications, offering comprehensive analytical solutions.
Key components of data analysis
Key components of data analysis outline the essential elements required for accurate data interpretation and modeling. These components include data cleaning, transformation, and statistical analysis techniques. The key characteristic of key components of data analysis is their role in streamlining the data analysis process and ensuring data quality. While their advantages encompass structured data presentation, the potential disadvantages may involve oversimplification or overlooking crucial data variables.
Objectives of Data Analysis
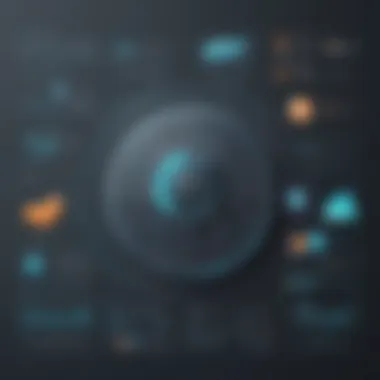
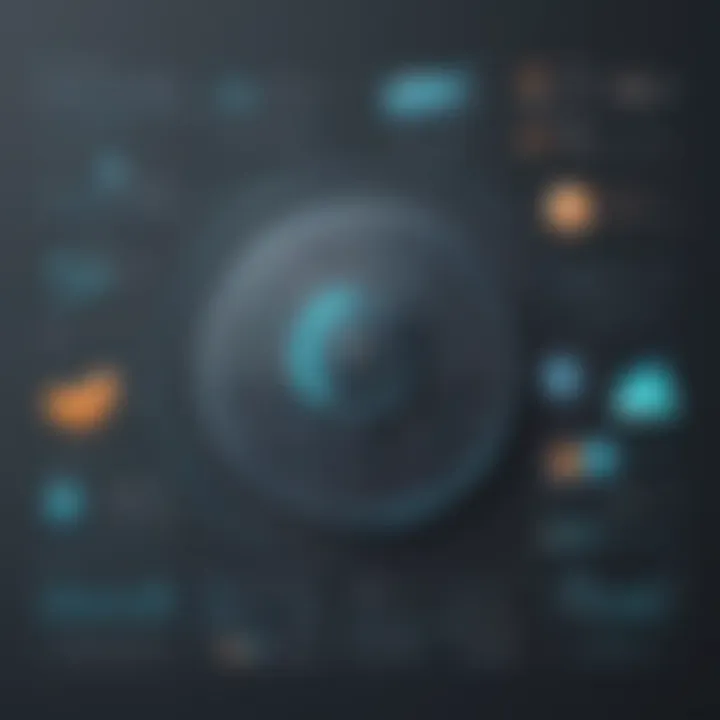
Exploring the goals of data analysis in research
Exploring the goals of data analysis in research involves identifying the primary objectives behind conducting data analysis. These goals may include hypothesis testing, pattern recognition, or predictive modeling. The key characteristic of exploring the goals of data analysis lies in aligning analytical strategies with research objectives to yield meaningful outcomes. Its unique feature is the ability to provide actionable insights for informed decision-making processes. While its advantages include data-driven predictions and trend identification, potential disadvantages may stem from data mismanagement or inadequate analytical tools.
Methods and Techniques of Data Analysis
Data analysis is the backbone of research, providing crucial insights into complex datasets. Understanding the methods and techniques used in data analysis is paramount for researchers and analysts alike. By delving into the nuances of quantitative data analysis, qualitative data analysis, and advanced data analysis techniques, a more profound understanding of data interpretation and pattern recognition can be achieved. This section will highlight the key points discussed in Methods and Techniques of Data Analysis, emphasizing their relevance in unlocking the depths of data analysis.
Quantitative Data Analysis
Quantitative data analysis involves the use of statistical methods to interpret numerical data systematically. Utilizing statistical methods is imperative in deriving meaningful insights from datasets, as it allows for the identification of patterns, trends, and correlations. The key characteristic of utilizing statistical methods lies in its ability to provide a quantitative foundation for research findings, enabling researchers to make data-driven decisions. Despite its widespread popularity, utilizing statistical methods also comes with limitations, such as the assumption of data normality and the need for large sample sizes. However, its advantages in terms of providing measurable and objective results make it a valuable choice for researchers seeking to quantify research outcomes.
Interpreting numerical data is another crucial aspect of quantitative data analysis. By deciphering the meaning behind numerical values, researchers can extract valuable information regarding the variables under study. The key characteristic of interpreting numerical data is its role in transforming raw numbers into actionable insights, aiding in hypothesis testing and result validation. While interpreting numerical data offers a structured approach to data analysis, it may also encounter challenges related to data outliers and skewed distributions. Nevertheless, its ability to provide concrete findings based on numeric values makes it a favorable option for researchers aiming to analyze quantitative data effectively.
Qualitative Data Analysis
In contrast to quantitative data analysis, qualitative data analysis focuses on exploring themes and patterns within textual or visual data. By delving into the narrative elements of datasets, researchers can uncover underlying meanings and contextual insights that quantitative analysis may overlook. The key characteristic of exploring themes and patterns is its emphasis on in-depth understanding and rich interpretation of data, allowing for subjective perspectives and contextualizations. Despite its interpretive nature, exploring themes and patterns may face challenges in terms of inter-coder reliability and researcher bias. Nonetheless, its advantage lies in providing a comprehensive narrative analysis that complements quantitative findings, offering a holistic view of research outcomes.
Conducting content analysis is a fundamental component of qualitative data analysis. By analyzing the content within textual or visual data, researchers can identify recurring themes, sentiments, and discourses prevalent within the dataset. The key characteristic of conducting content analysis is its structured approach towards categorizing and interpreting data content, facilitating a systematic examination of qualitative information. While conducting content analysis enhances data organization and content deconstruction, it may also encounter issues of subjectivity and theme misinterpretation. Nevertheless, its ability to extract meaningful insights from qualitative data makes it an indispensable tool for researchers seeking to explore the intricacies of textual and visual information.
Advanced Data Analysis Techniques
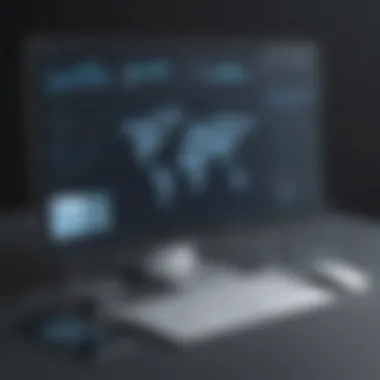
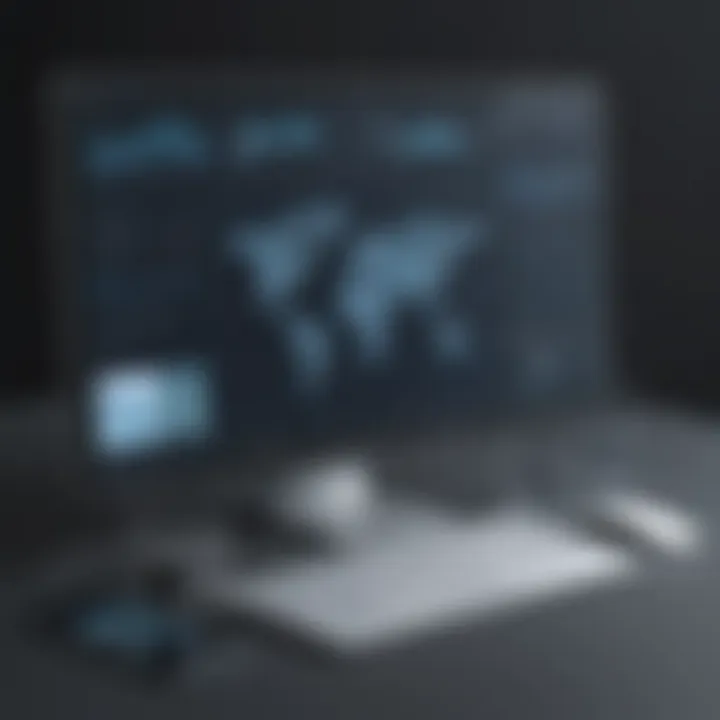
Advanced data analysis techniques encompass sophisticated methodologies such as machine learning algorithms and predictive modeling. These techniques enable researchers to uncover intricate patterns and relationships within datasets, offering predictive capabilities and trend forecasting. Machine learning algorithms showcase a distinctive feature by leveraging computational algorithms to iteratively learn from data and make predictions without explicit programming. This unique feature empowers researchers to handle large and complex datasets efficiently, leading to accurate predictions and data classifications. Despite the complexities associated with machine learning algorithms in terms of model interpretability and overfitting, their advantages in automating data analysis processes and enhancing accuracy establish them as a prominent choice for researchers seeking actionable insights from extensive datasets.
Predictive modeling, on the other hand, focuses on developing predictive models based on existing data to forecast future trends or outcomes. The key characteristic of predictive modeling lies in its ability to extrapolate patterns and dependencies within data to predict potential scenarios or behaviors. By employing statistical methodologies and analytical tools, researchers can construct predictive models that aid in decision-making and risk assessment. While predictive modeling offers foresight into future trends, its limitations may include over-reliance on historical data and assumptions that can impact the accuracy of predictions. Nonetheless, its advantages in identifying patterns and trends in data, making it invaluable for researchers exploring predictive analytics to support informed decision-making processes.
Significance of Data Analysis in Research
Data analysis holds paramount significance in the realm of research, acting as a linchpin that anchors the validity and reliability of research findings. Within the multifaceted landscape of data analysis, its role transcends mere number-crunching to becoming a conduit for informed decision-making and meaningful interpretation of research outcomes. By meticulously probing and unraveling the intricate layers of datasets, researchers can extract invaluable insights that drive the narrative of their studies. The essence of data analysis lies in its ability to transform raw data points into actionable intelligence, empowering researchers to draw evidence-based conclusions that underpin the essence of scientific inquiry. Through a systematic and strategic approach, data analysis not only elucidates trends and patterns but also enhances the overall credibility and accuracy of research endeavors.
Challenges and Limitations of Data Analysis
In the realm of data analysis, confronting challenges and limitations is a crucial aspect that cannot be overlooked. This segment of the comprehensive guide aims to shed light on the significance of understanding and overcoming these obstacles in the data analysis process. By recognizing and addressing these challenges, researchers and analysts can enhance the quality and reliability of their insights, thereby fostering more accurate decision-making and research outcomes.
Overcoming Data Complexity
Addressing Data Quality Issues
Delving into the intricacies of data quality is paramount when unraveling the layers of data complexity within analytical projects. Effectively managing and rectifying data quality issues ensures the integrity and trustworthiness of analysis outcomes. By meticulously scrutinizing data quality parameters such as accuracy, completeness, consistency, and validity, analysts can mitigate errors and biases, thereby bolstering the credibility of their findings. Being vigilant in addressing data quality concerns not only refines the accuracy of interpretations but also cultivates a robust foundation for subsequent analyses, fostering a more efficient and effective research process.
Managing Large Datasets
Navigating the vast sea of data encompassed in large datasets presents a formidable challenge for analysts. Managing massive volumes of data requires strategic planning, robust infrastructure, and sophisticated tools to streamline storage, retrieval, and analysis procedures. By implementing advanced techniques like data partitioning, indexing, and parallel processing, analysts can optimize data management practices, ensuring efficient processing and accurate results. Despite the complexities inherent in handling large datasets, adept management not only accelerates decision-making processes but also unveils valuable insights that may remain hidden in smaller-scale analyses, elevating the scope and depth of research endeavors.
Interpreting Ambiguous Results
Grasping the complexities of ambiguous results is a critical skill in the realm of data analysis, requiring analysts to navigate uncertainties with precision and diligence. Uncertainty is an inherent component of data analysis, stemming from various sources such as data variability, measurement errors, and sampling biases. Navigating through these uncertainties demands a nuanced approach that incorporates statistical techniques, sensitivity analyses, and expert judgment to arrive at robust conclusions. By embracing uncertainty as a catalyst for deeper exploration and refinement of analytical methods, analysts can transform ambiguous outcomes into opportunities for enhancing the depth and reliability of their research findings.
Ethical Considerations in Data Analysis
Amidst the data-driven landscape of analysis, ethical considerations emerge as a critical cornerstone that underscores the integrity and trustworthiness of research endeavors. Ensuring data privacy and confidentiality is not just a legal obligation but a moral imperative that safeguards the rights and interests of individuals whose data is being analyzed. By adhering to stringent privacy policies, robust encryption measures, and secure data handling protocols, analysts demonstrate respect for data subjects' autonomy and confidentiality, fostering a culture of transparency and trust in their analytical practices. Prioritizing ethical standards in data analysis not only upholds the reputation and credibility of researchers but also cultivates a conducive environment for responsible and ethical data utilization, fostering a sustainable and resilient research ecosystem.