Unlocking the Profound Potential of Data Analytics Through Big Data Deciphering
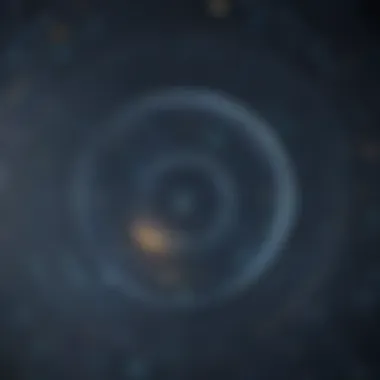
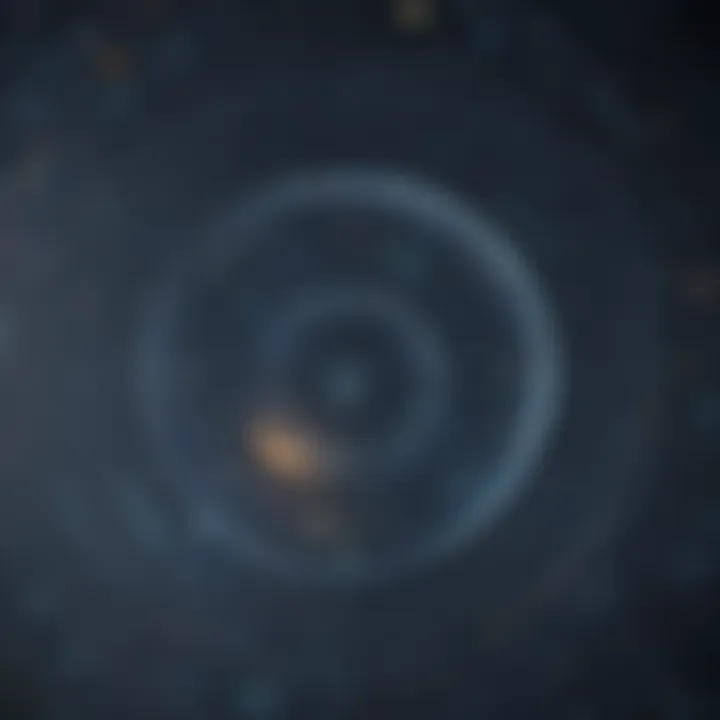
Overview of Big Data and Data Analytics
Big data and data analytics are pivotal concepts reshaping industries worldwide. This section encapsulates the essence of these transformative technologies, underlining their profound impact on innovation and decision-making across diverse sectors. Explore the origins and evolvement of big data, delving into its evolution alongside data analytics.
Fundamentals Deciphered
Dive into the foundational principles and core theories surrounding big data and data analytics. Unravel key terminologies and meticulous definitions crucial for comprehending the intricacies of these fields. Grasp the fundamental concepts and foundational knowledge that form the backbone of big data and data analytics.
Practical Implementation and Exemplars
Immerse yourself in real-world case studies and practical applications to uncover the practicality of big data and data analytics. Engage with demonstrations and hands-on projects that shed light on the nuances of utilizing these technologies. Gain insights into coding snippets and implementation guidelines for practical application.
Advanced Insights and Emerging Trends
Stay abreast of cutting-edge developments in the domain of big data and data analytics. Explore advanced techniques, methodologies, and tools that are shaping the future of data analytics. Peer into the future prospects and upcoming trends that are set to revolutionize these fields.
Recommendations for Further Learning
Navigate through recommended books, courses, and online resources tailored for enthusiasts, students, and professionals eager to delve deeper into big data and data analytics. Discover essential tools and software conducive to enhancing practical usage and implementation in various projects.
Understanding Big Data
In this section of the article, we will delve into the crucial concept of Understanding Big Data. Big Data plays a pivotal role in reshaping the landscape of data analytics across various industries. It is imperative to comprehend the intricate elements, benefits, and considerations associated with Understanding Big Data to harness its full potential.
Defining Big Data
Volume
Exploring the aspect of Volume within the realm of Big Data is paramount to grasping its magnitude. Volume refers to the vast amount of data generated daily from diverse sources like social media, sensors, and transactions. The key characteristic of Volume lies in the substantial amount of information collected, providing a rich source for analysis. The unique feature of Volume is its capacity to reveal patterns and trends that might otherwise remain hidden. Despite its advantages in revealing valuable insights, managing and processing voluminous data can pose challenges in terms of storage and processing capabilities.
Variety
The Variety aspect of Big Data showcases the diverse nature of data types available for analysis. Ranging from structured to unstructured data, Variety encompasses text, images, videos, and more. This diversity enables a comprehensive understanding of different data sources, enriching the insights derived from analysis. The key characteristic of Variety lies in its ability to capture information from multiple channels, offering a holistic view of the data landscape. While Variety enhances the richness of data interpretation, handling disparate data formats can complicate the analysis process.
Velocity
Velocity highlights the speed at which data is generated and processed in real-time. With the advent of IoT devices and digital platforms, data is produced at an unprecedented pace. This rapid flow of data empowers organizations to make timely decisions based on up-to-date information. The key characteristic of Velocity is its agility in providing immediate insights for swift action. However, managing data streams at high speeds can strain existing infrastructure and require robust processing mechanisms to keep up with the influx of information.
Importance of Big Data
The significance of Big Data cannot be overstated, especially in the context of data analytics. It serves as the driving force behind Insight Generation, Predictive Analytics, and Enhanced Decision Making. These key aspects leverage the power of data to uncover valuable information, forecast future trends, and facilitate informed decision-making processes.
Insight Generation
Insight Generation involves mining data to extract meaningful information that informs strategic decisions. By analyzing patterns and correlations within data sets, organizations can gain valuable insights into consumer behavior, market trends, and operational efficiencies. The key characteristic of Insight Generation is its ability to transform raw data into actionable insights, enabling businesses to adapt and thrive in dynamic environments.
Predictive Analytics
Predictive Analytics utilizes historical data to forecast future outcomes and trends. By employing advanced algorithms and statistical models, organizations can anticipate market fluctuations, customer preferences, and potential risks. The key characteristic of Predictive Analytics is its capacity to enhance proactive decision-making based on data-driven predictions. While offering valuable foresight, predictive models require accurate and comprehensive data inputs to ensure reliable forecasts.
Enhanced Decision Making
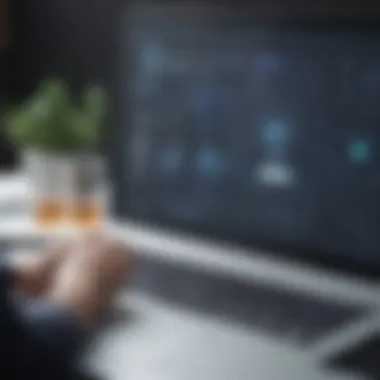
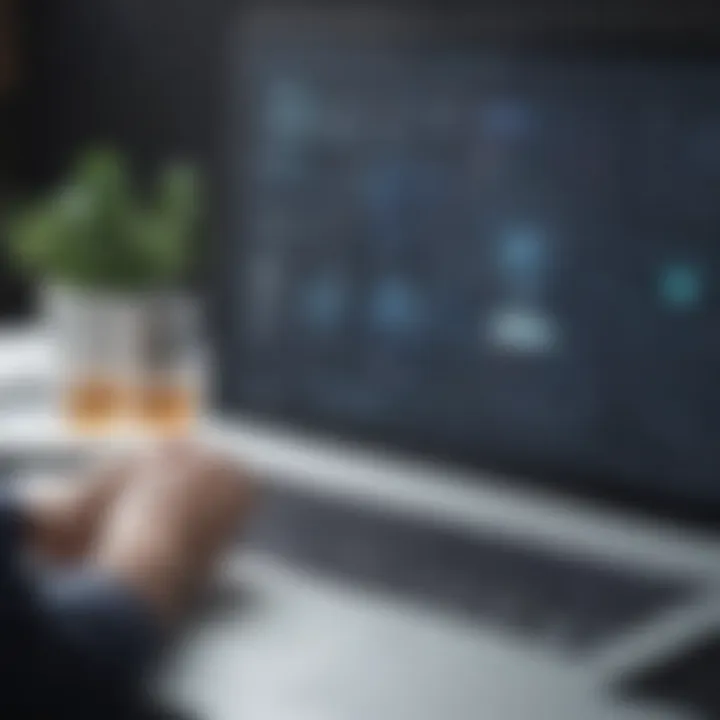
Enhanced Decision Making integrates data-driven insights into the decision-making process, empowering stakeholders with relevant information for strategic choices. By incorporating data analysis techniques, organizations can optimize resource allocation, mitigate risks, and identify growth opportunities. The key characteristic of Enhanced Decision Making is its ability to augment human judgment with data-backed evidence, catalyzing informed and effective decision-making. However, balancing data-driven decisions with qualitative considerations is essential to ensure comprehensive and holistic outcomes.
Characteristics of Big Data
The Characteristicsof Big Data, including Scale, Complexity, and Accuracy, define the nature of data sets and influence analytics outcomes.'Scale 'reflects the vastness of data volume, 'Complexity' signifies the diversity of data types, and 'Accuracy' underscores the precision of data interpretations. These characteristics collectively shape the landscape of Big Data analytics, presenting opportunities and challenges for organizations seeking to harness data's potential for innovation and growth.
Scale
Scaling refers to the ability to manage and analyze massive volumes of data effectively. As data continues to proliferate, organizations must employ scalable solutions to accommodate growing data sets without sacrificing performance. The key characteristic of Scale is its capacity to handle large volumes of data to extract valuable insights efficiently. While scalability boosts analytical capabilities, it necessitates robust infrastructure and data management strategies to support seamless operations.
Complexity
The Complexity of Big Data pertains to the intricate nature of data sources and formats encountered in analytics projects. From structured data in databases to unstructured data in social media feeds, Complexity underscores the varied forms data can assume. The key characteristic of Complexity lies in the diverse data landscapes it encompasses, offering a multi-faceted view of information for analysis. However, navigating the complexities of disparate data structures requires sophisticated tools and expertise to derive coherent insights effectively.
Accuracy
Accuracy plays a crucial role in ensuring the reliability and precision of data analytics results. Data accuracy is vital for making informed decisions and drawing valid conclusions from analysis outcomes. The key characteristic of Accuracy is its emphasis on delivering trustworthy insights that align with business objectives and operational requirements. Maintaining data accuracy involves rigorous validation processes and quality assurance measures to enhance the credibility and utility of analytical findings.
Applications of Big Data
Big Data applications play a pivotal role in leveraging datasets for actionable insights. In this article, we delve into the diverse applications of Big Data across various industries, shedding light on its transformative capabilities. By harnessing Big Data, organizations can enhance decision-making processes, refine operations, and gain competitive advantages in the market. The extensive utilization of data analytics tools allows for a deeper understanding of consumer behaviors, market trends, and operational efficiencies.
In Business
Market Analysis
Market Analysis stands as a cornerstone in optimizing business strategies. This analytical approach provides valuable insights into market dynamics, consumer preferences, and competitor activities. Leveraging Big Data for Market Analysis enables businesses to identify growth opportunities, forecast demand, and adjust marketing strategies for maximum impact. Despite its complexity, Market Analysis offers a holistic view of the market landscape, empowering companies to make informed decisions swiftly.
Customer Insights
Customer Insights extracted from Big Data analytics offer a wealth of information regarding consumer preferences, buying patterns, and brand interactions. By analyzing customer data, businesses can tailor products and services to meet specific needs, enhance customer experiences, and foster long-term relationships. Understanding Customer Insights aids in creating targeted marketing campaigns, improving customer retention, and ultimately driving revenue growth.
Operational Efficiency
Streamlining operational processes through data-driven insights is imperative for sustainable business growth. Operational Efficiency involves optimizing resource allocation, workflow management, and performance metrics using Big Data analytics. By identifying inefficiencies, redundancies, and bottlenecks, organizations can enhance productivity, reduce costs, and enhance overall operational effectiveness. Implementing data-driven strategies improves workflow efficiency and enables proactive decision-making to drive operational excellence.
In Healthcare
Patient Care
Enhancing Patient Care through Big Data analytics revolutionizes healthcare services by personalizing treatment plans, improving diagnostics, and predicting patient outcomes. By leveraging patient data, healthcare providers can offer customized care, reduce hospital readmission rates, and optimize treatment protocols. Implementing data-driven approaches in Patient Care not only enhances healthcare quality but also increases patient satisfaction and promotes better health outcomes.
Disease Prevention
Big Data analytics plays a critical role in Disease Prevention by identifying risk factors, detecting outbreaks, and tracking trends in public health. By analyzing population health data, healthcare organizations can implement preventive measures, develop vaccination strategies, and mitigate the spread of infectious diseases. Disease Prevention strategies powered by Big Data ensure proactive healthcare interventions, reduce healthcare costs, and improve overall community health.
Medical Research
Innovations in Medical Research are fueled by Big Data analytics, enabling accelerated drug discovery, clinical trials optimization, and precision medicine advancements. Utilizing vast datasets, researchers can identify disease patterns, explore treatment effectiveness, and uncover new therapeutic targets. By incorporating data-driven methodologies, Medical Research can push the boundaries of scientific knowledge, drive medical breakthroughs, and improve healthcare outcomes for individuals and populations.
In Finance
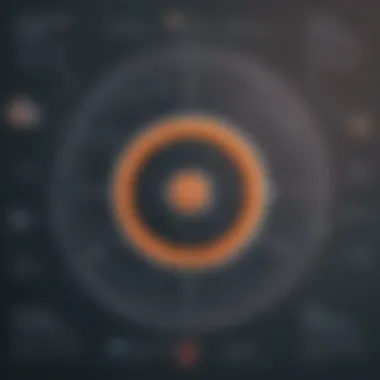
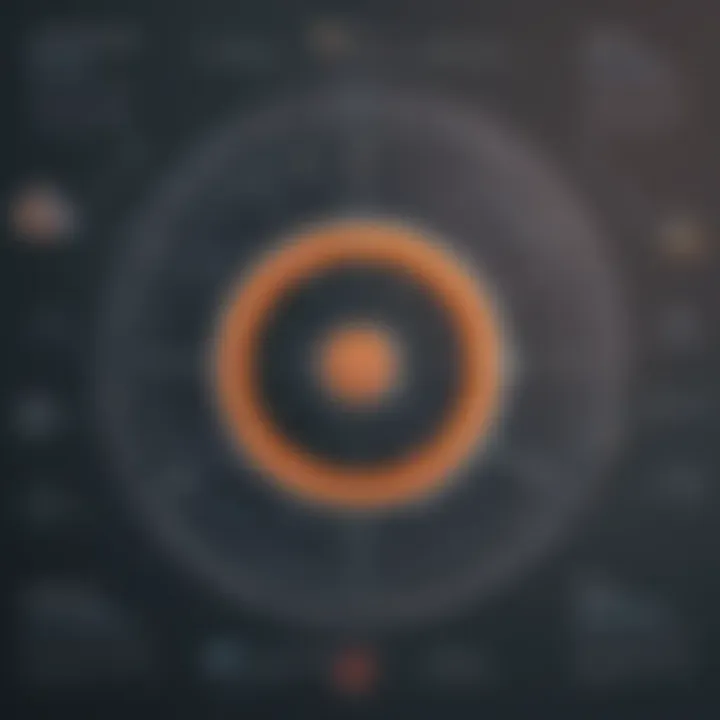
Risk Management
Efficient Risk Management practices rely on Big Data analytics to identify, assess, and mitigate financial risks in various sectors. By analyzing market trends, financial indicators, and risk factors, organizations can proactively manage exposures, optimize risk-return profiles, and comply with regulatory requirements. Implementing comprehensive Risk Management strategies supported by Big Data enhances decision-making processes, protects investments, and ensures financial sustainability.
Fraud Detection
Fraud Detection mechanisms powered by Big Data analytics help financial institutions combat fraudulent activities, detect anomalies, and prevent financial crimes. By utilizing machine learning algorithms, predictive models, and transaction monitoring systems, organizations can detect suspicious behavior, investigate fraud cases, and safeguard sensitive financial information. Integrating advanced Fraud Detection tools enhances fraud prevention capabilities, safeguards consumer trust, and minimizes financial losses.
Trading Strategies
Optimizing Trading Strategies through Big Data analytics enables investors to make data-driven investment decisions, forecast market trends, and maximize returns on investments. By analyzing historical data, market signals, and trading patterns, traders can develop algorithmic trading models, risk management strategies, and portfolio optimization techniques. Leveraging Big Data for Trading Strategies empowers investors to capitalize on market opportunities, mitigate risks, and achieve investment objectives efficiently.
Challenges of Big Data
Big data presents numerous challenges that must be addressed for effective utilization. Understanding these challenges is crucial for businesses and organizations aiming to harness the power of data analytics. The complexities within big data highlight the need for tailored solutions to ensure data-driven insights lead to informed decisions. One key area of concern is data privacy, which encompasses security risks, compliance issues, and ethical considerations.
Data Privacy
Data privacy is paramount in the era of big data, where vast amounts of sensitive information are collected, stored, and analyzed. The protection of data against unauthorized access and use is critical to maintaining trust and complying with regulations. Within the realm of data privacy lie three significant aspects: security risks, compliance issues, and ethical concerns.
Security Risks
Security risks within data privacy pertain to the vulnerabilities that data faces from potential breaches. These risks arise from cyber threats, hacker attacks, or internal leaks, posing a substantial threat to the confidentiality and integrity of data. Understanding the nature of these security risks is vital in implementing robust security measures to safeguard data assets effectively.
Compliance Issues
Compliance issues revolve around adhering to regulatory requirements and industry standards concerning data protection and privacy. Non-compliance can result in severe legal ramifications and damage to an organization's reputation. By addressing compliance issues proactively, businesses can ensure that their data practices align with legal frameworks and best practices within the industry.
Ethical Concerns
Ethical considerations in data privacy focus on the moral implications of data collection, usage, and processing. This includes aspects such as informed consent, data transparency, and fairness in algorithmic decision-making. Ethical dilemmas in big data require a careful balance between innovation and responsible data handling to uphold societal values and individual rights.
Data Quality
Ensuring the quality of data is essential for deriving accurate and reliable insights. Data quality encompasses factors such as accuracy, consistency, and completeness, which directly impact the effectiveness of analytical outcomes. Addressing data quality concerns involves refining data collection processes, implementing validation mechanisms, and maintaining data integrity.
Accuracy
The accuracy of data refers to its correctness and precision in relation to the real-world entities or phenomena it represents. High data accuracy ensures that analytical results are trustworthy and align with the intended objectives. Establishing data accuracy involves validation procedures, error detection, and data cleansing techniques to minimize discrepancies and inaccuracies.
Consistency
Data consistency pertains to the uniformity and coherence of data across different sources, formats, and timeframes. Consistent data sets enable comparative analysis, trend identification, and reliable decision-making. Maintaining data consistency involves data integration, normalization, and synchronization to harmonize disparate data elements into a cohesive data repository.
Completeness
Data completeness signifies the presence of all required data fields and values within a dataset. Incomplete data can lead to biased insights, flawed conclusions, and inaccurate predictions. Enhancing data completeness entails data profiling, quality checks, and data augmentation strategies to fill gaps and ensure comprehensive data coverage.
Infrastructure
A robust data infrastructure is fundamental for handling the volume and complexity of big data efficiently. Infrastructure requirements encompass scalability, storage capabilities, and processing power to support the diverse analytical needs of organizations. Building a resilient data infrastructure involves deploying scalable architectures, advanced storage solutions, and high-performance computing resources.
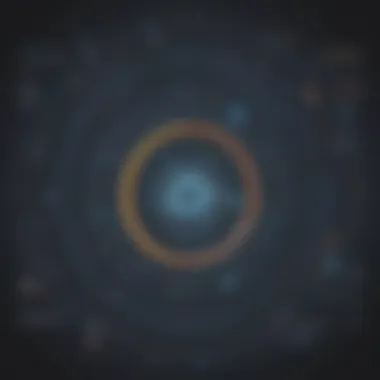
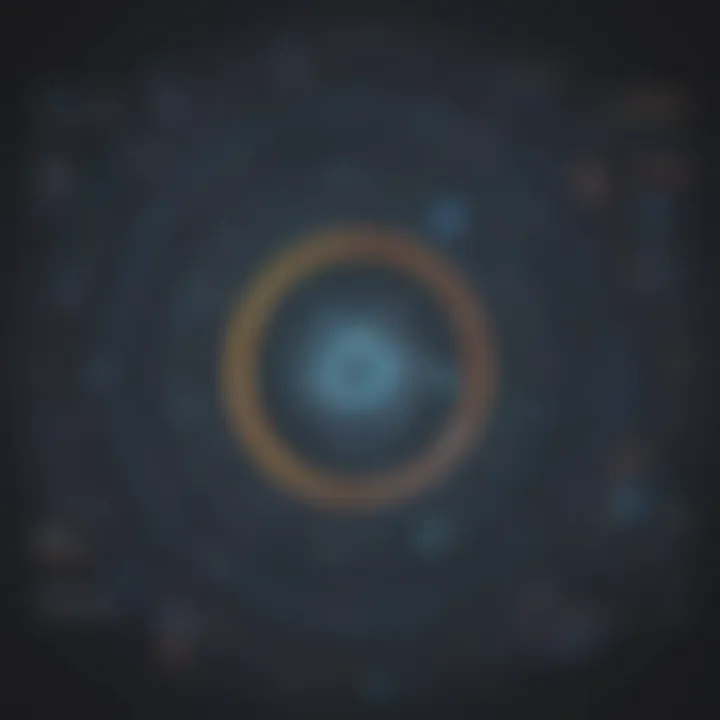
Scalability
Scalability in data infrastructure refers to the ability to expand system capacities seamlessly as data volumes and processing demands increase. Scalable architectures enable organizations to accommodate growing data loads without compromising performance or reliability. Implementing scalable solutions involves modular design, cloud integration, and resource elasticity to scale infrastructure components dynamically.
Storage
Efficient data storage is essential for securely housing massive datasets and ensuring data accessibility for analytics processes. Storage solutions must optimize data retrieval, retention, and archival processes while facilitating data sharing and collaboration. Selecting appropriate storage technologies based on data characteristics and usage patterns is crucial for meeting data storage requirements effectively.
Processing Power
The processing power of data infrastructure influences the speed and efficiency of data processing tasks, such as real-time analytics, batch processing, and machine learning algorithms. High processing power enhances data processing capabilities, enabling rapid decision-making, complex calculations, and data transformations. Enhancing processing power entails deploying specialized processors, parallel processing techniques, and optimized algorithms for maximizing computational performance.
Future Trends in Big Data
In this article, we delve into the crucial aspect of future trends in big data. Understanding the evolving landscape of data analytics is pivotal for staying ahead in various industries. Embracing the power of future trends in big data empowers organizations to harness advanced technologies for improved decision-making. By discussing machine learning, IoT integration, and blockchain technology, we shed light on transformative trends reshaping data analytics.
Machine Learning
Predictive Modeling
Predictive modeling stands as a cornerstone in the realm of data science, offering predictive capabilities based on historical data patterns. Its ability to forecast future trends and outcomes fuels informed decision-making processes. The key characteristic of predictive modeling lies in its utilization of algorithms to analyze data and predict future trends accurately. This analytical approach is a preferred choice in this article as it enables organizations to anticipate market trends and customer behavior effectively. Despite its efficacy, predictive modeling may face challenges in handling complex data relationships and ensuring model accuracy.
Natural Language Processing
Natural Language Processing (NLP) plays a pivotal role in extracting insights from unstructured textual data. Its ability to process human language enables sentiment analysis, language translation, and text summarization, contributing significantly to data analytics. The key characteristic of NLP is its capability to interpret and generate human-like text, enhancing data processing efficiency. NLP's unique feature lies in its adaptability across various domains, making it a versatile tool for text analysis in this article. However, challenges such as context ambiguity and language nuances may impact NLP's accuracy and performance.
Recommendation Systems
Recommendation systems are instrumental in personalized user experiences by suggesting products or content based on user preferences. Their key characteristic lies in algorithmic prediction of user choices, enhancing user engagement and satisfaction. In this article, recommendation systems serve to optimize customer interactions and drive sales through targeted recommendations. The unique feature of recommendation systems is their ability to enhance user engagement and retention through personalized suggestions. Although effective, challenges such as data privacy concerns and algorithm biases require careful consideration in deploying recommendation systems.
IoT Integration
Sensor Data
Sensor data captures real-world information through interconnected devices, enabling real-time analytics and enhanced decision-making. The key characteristic of sensor data lies in its ability to collect diverse data types from IoT devices, providing valuable insights for businesses. Sensor data's unique feature includes its role in enabling predictive maintenance and operational efficiency improvements in this article. Despite its advantages, challenges like data security and interoperability issues pose risks to sensor data integration.
Real-time Insights
Real-time insights offer actionable data analysis and immediate responses to changing circumstances, providing a competitive edge in dynamic environments. The key characteristic of real-time insights is timely data processing, empowering organizations to make agile decisions based on up-to-date information. Real-time insights' unique feature lies in their ability to drive proactive strategies and optimize operational processes in this article. However, issues such as data latency and technological limitations may hinder the accuracy and reliability of real-time insights.
Automation
Automation streamlines repetitive tasks and processes through technological solutions, improving operational efficiency and reducing manual errors. The key characteristic of automation is its ability to accelerate task completion and optimize resource utilization, enhancing organizational productivity. Automation's unique feature lies in its adaptability across industries, offering cost-effective solutions for workflow optimization in this article. Despite its benefits, challenges such as job displacement and system vulnerabilities require proactive risk management in implementing automation solutions.
Blockchain Technology
Enhanced Security
Enhanced security in blockchain technology involves cryptographic protection mechanisms, ensuring data integrity and confidentiality. The key characteristic of enhanced security is its decentralized nature, preventing single points of failure and unauthorized data access. In this article, enhanced security through blockchain technology enhances data protection and reduces cybersecurity risks. The unique feature of enhanced security lies in its transparent and tamper-resistant data storage, mitigating security breaches and unauthorized data modifications. However, scalability challenges and regulatory compliance issues demand ongoing enhancements to maximize the benefits of enhanced security protocols.
Immutable Records
Immutable records in blockchain technology facilitate transparent and secure transaction records through cryptographic algorithms. The key characteristic of immutable records is their unchangeable nature, providing verifiable transaction histories and audit trails. Immutable records play a pivotal role in this article by ensuring data integrity and auditability across interconnected systems. The unique feature of immutable records lies in their ability to establish trust and transparency in transaction processes, minimizing fraud risks and data tampering. However, concerns surrounding privacy regulations and data governance may impact the adoption and implementation of immutable record systems.
Smart Contracts
Smart contracts are self-executing agreements based on predefined rules and conditions encoded within blockchain networks. The key characteristic of smart contracts is their automation of contract enforcement and transaction settlements without intermediary involvement. Smart contracts offer efficiency gains and reduced costs in this article by automating contractual processes and mitigating disputes through code-based execution. The unique feature of smart contracts lies in their transparency and auditability, ensuring parties adhere to agreed terms without the need for legal intermediaries. Despite their advantages, challenges such as code vulnerabilities and regulatory uncertainties require tailored smart contract development and legal validations for seamless integration.