Unveiling Insights: Visualizing Big Data Through Data Representation
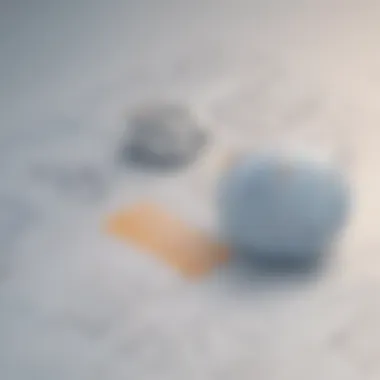
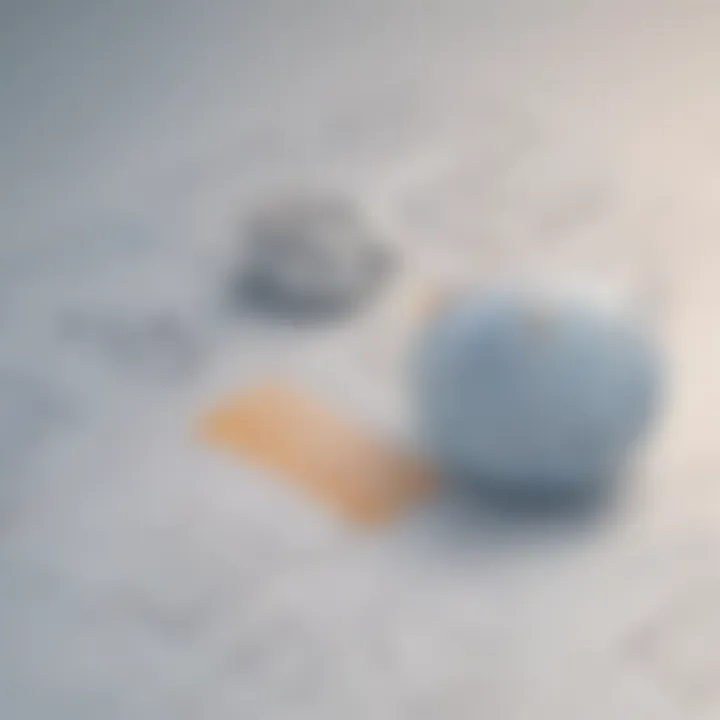
In this section, we delve into the intricate process of visualizing large datasets, unlocking hidden insights through innovative data representation methods. The significance of data visualization in deciphering complex datasets is paramount in today's tech-driven world. By understanding visualization techniques and their impact on decision-making, we can transform raw data into actionable insights.
Fundamentals Explained
In the Fundamentals Explained section, we explore the core principles and theories related to visualizing big data. Key terminology and definitions are clarified to establish a solid foundational knowledge base for readers. By delving into basic concepts, individuals can grasp the essence of data representation in a simplified manner, aiding in the comprehension of more complex topics that follow.
Practical Applications and Examples
Real-world case studies and applications are crucial in understanding the practical implications of visualizing big data. Through demonstrations and hands-on projects, readers can experience firsthand the power of data representation. Code snippets and implementation guidelines provide a practical approach, allowing individuals to apply theoretical knowledge to concrete scenarios.
Advanced Topics and Latest Trends
Exploring cutting-edge developments in the field of visualizing big data sheds light on advanced techniques and methodologies. The evolution of data representation unveils future prospects and upcoming trends, highlighting the perpetual innovation within this critical domain. By contemplating these advanced topics, readers gain insight into the dynamic nature of big data visualisation.
Tips and Resources for Further Learning
For those seeking to deepen their understanding, recommended books, courses, and online resources serve as invaluable tools. Access to tools and software suited for practical usage equips individuals with the necessary resources to explore visualizing big data further in their professional journeys. By providing ample resources for further learning, this section aims to empower readers in their pursuit of mastering data representation.
Introduction
Visualizing big data plays a pivotal role in modern data analysis, offering profound insights into complex datasets through innovative data representations. In this article, we delve deep into the realm of data visualization, exploring its significance in deciphering intricate data structures and extracting actionable insights that drive informed decision-making.
Defining Big Data Visualization
In the realm of data analytics, understanding the concept of big data visualization is paramount. By leveraging advanced visualization techniques, organizations can gain a comprehensive understanding of vast data sets, enabling them to uncover valuable patterns and trends that may otherwise remain hidden. The process of visualizing big data involves transforming raw information into visual representations that are easily interpretable, enhancing data comprehension and paving the way for data-driven decisions. Embracing big data visualization is instrumental in unraveling the complexities of modern data landscapes and harnessing the power of visual storytelling.
Understanding the Concept of Big Data
When delving into the concept of big data visualization, it becomes apparent that its primary goal is to make large and complex data sets more accessible and understandable. By creating visual representations of data, organizations can synthesize information efficiently, identify correlations, and extract meaningful insights at a glance. This approach serves as a gateway to unlocking the potential hidden within vast data repositories, offering a visual roadmap for data exploration and analysis.
Importance of Visualizing Big Data
The importance of visualizing big data lies in its ability to transform raw numbers into compelling visual narratives. By harnessing the power of data visualization, organizations can communicate data-driven insights effectively, fostering a deeper understanding of complex data sets across various stakeholders. Visual representations offer a more intuitive way of processing information, enabling quicker decision-making and promoting data-driven strategies that align with organizational objectives.
Benefits of Effective Data Visualization
Effective data visualization brings a multitude of benefits to the table, ranging from enhanced data comprehension to improved decision-making processes. By presenting data in visually engaging formats, organizations can streamline communication, streamline complex information, and reveal underlying patterns and trends that drive actionable insights. The visual clarity offered by effective data visualization empowers users to grasp complex concepts effortlessly, fostering a culture of data literacy and strategic decision-making.
Significance of Data Representation
The significance of data representation transcends mere visual aesthetics, playing a fundamental role in enhancing data comprehension, facilitating decision-making processes, and identifying patterns and trends critical to organizational success. Through interactive and insightful data representations, stakeholders can unravel the complexities of data landscapes, make informed decisions, and gain a competitive edge by leveraging data-driven strategies.
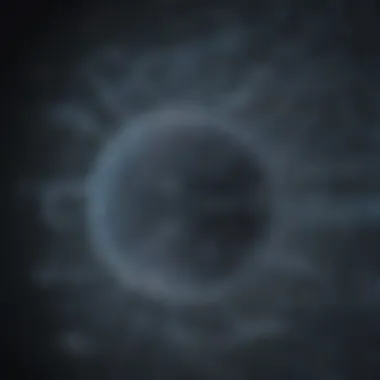
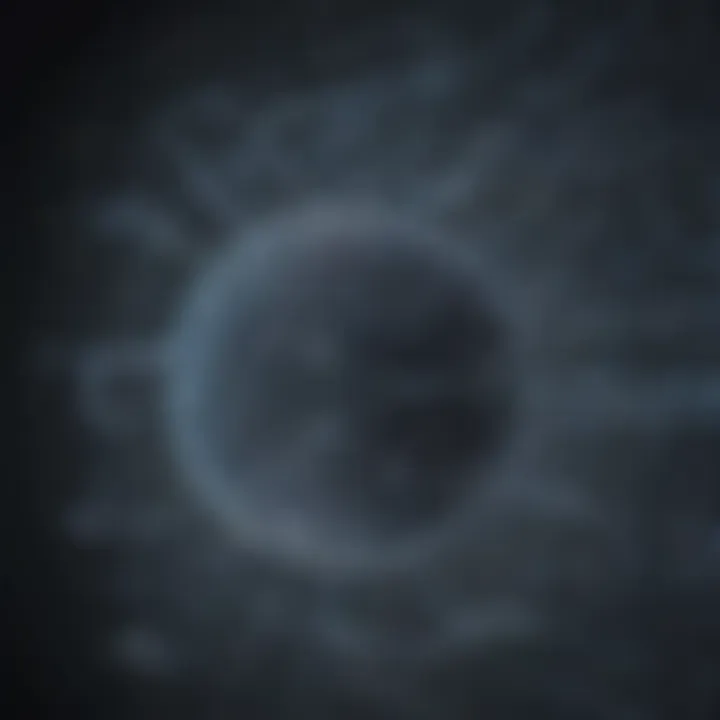
Enhancing Data Comprehension
Enhancing data comprehension through effective data representation is essential in navigating the intricate web of complex data sets. By presenting data in a visually coherent manner, organizations can simplify information, highlight critical insights, and propel data-driven narratives that inform strategic decision-making processes. Effective data visualization acts as a conduit for knowledge transfer, enabling stakeholders to grasp complex data relationships and make informed choices based on actionable insights.
Facilitating Decision-Making Processes
Facilitating decision-making processes through data representation empowers organizations to make informed and strategic choices based on data insights. By visually capturing key data metrics and trends, decision-makers can evaluate potential outcomes, assess risks, and devise informed strategies that align with organizational goals. Data-driven decision-making facilitated by compelling data representations forms the bedrock of agile and adaptive organizational practices, setting the stage for sustained growth and innovation.
Identifying Patterns and Trends
Identifying patterns and trends within vast data sets is a cornerstone of effective data representation strategies. By leveraging visualizations to spot anomalies, correlations, and predictive trends, organizations can gain invaluable insights into market dynamics, consumer behavior, and operational performance. Unveiling patterns and trends through data representation equips organizations with the foresight to anticipate future trends, mitigate risks, and capitalize on emerging opportunities, making data-driven decisions that drive sustainable growth.
Techniques for Visualizing Big Data
Visualizing big data is a critical aspect of deriving actionable insights from complex datasets. In this article, we focus on exploring various techniques that play a pivotal role in making data more comprehensible and facilitating informed decision-making processes. Through a strategic approach to data visualization, businesses can uncover hidden patterns, trends, and correlations, transforming raw data into valuable information that drives strategic initiatives and enhances overall operational efficiency.
Interactive Data Visualization
Utilizing Dashboards and Heatmaps
Utilizing dashboards and heatmaps is a fundamental aspect of interactive data visualization that offers a comprehensive overview of key metrics and trends. Dashboards provide a centralized platform for monitoring real-time data, enabling users to track performance indicators and KPIs effectively. Heatmaps, on the other hand, provide a visual representation of data density, making it easier to identify areas of interest or anomalies within large datasets. The integration of dashboards and heatmaps enhances the user experience by presenting information in a visually compelling manner, promoting better data analysis and interpretation.
Implementing Drill-Down Features
Implementing drill-down features allows users to delve deeper into specific data points or subsets, providing a more detailed view of the underlying information. This functionality enables analysts to explore data hierarchies and relationships, uncovering valuable insights that may not be apparent at a surface level. By allowing for interactive exploration of data layers, drill-down features empower users to extract meaningful conclusions and make informed decisions based on a comprehensive understanding of the dataset.
Real-Time Data Interaction
Real-time data interaction is a dynamic feature that enables users to engage with data instantaneously, capturing and responding to updates in real-time. This functionality is particularly valuable in fast-paced environments where timely decision-making is crucial. By facilitating immediate access to up-to-date information, real-time data interaction enhances the agility and responsiveness of businesses, enabling stakeholders to act swiftly on emerging opportunities or threats.
Infographics and Data Visualizations
Creating Engaging Visual Representations
Creating engaging visual representations involves developing compelling infographics that convey complex information in a clear and visually appealing manner. Infographics combine data visualizations with concise text and graphic elements to communicate key messages effectively. By employing visually stimulating design elements, infographics capture the audience's attention and facilitate better retention of information, making them an ideal choice for presenting key insights in a digestible format.
Summarizing Complex Data Sets
Summarizing complex data sets through visual representations aids in distilling voluminous information into easy-to-understand summaries. By condensing large datasets into concise visual components, complex relationships and patterns become readily apparent, enabling stakeholders to grasp essential insights at a glance. This approach simplifies data interpretation and communication, enhancing the overall accessibility and utility of information for decision-making purposes.
Enhancing Data Communication
Enhancing data communication involves leveraging visual aids to convey information in a compelling and accessible way. By using data visualizations to tell a story or present key findings, organizations can improve the effectiveness of their data communication strategies. Visual representations enhance engagement, promote data-driven discussions, and foster a more profound understanding among diverse stakeholders, ultimately supporting collaborative decision-making processes.
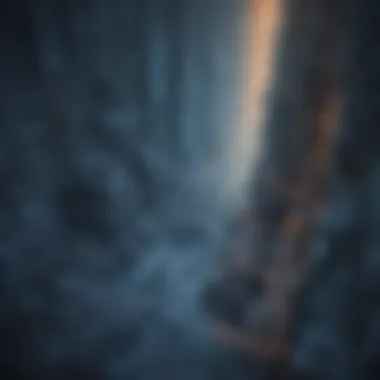
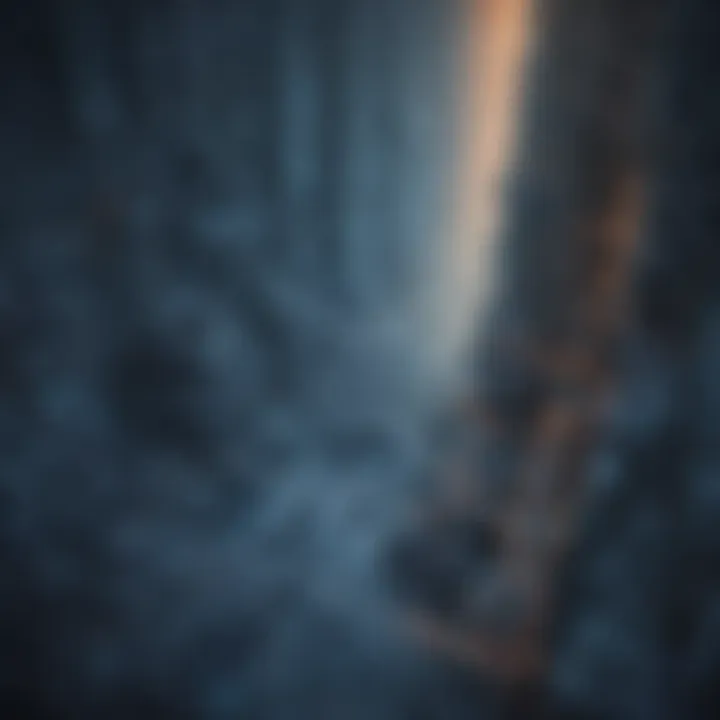
Geospatial Data Visualization
Mapping Data for Geographic Insights
Mapping data for geographic insights involves visualizing data points on maps to identify spatial trends and patterns. By geographically mapping datasets, businesses can pinpoint location-based correlations, distributions, and clusters, gaining valuable insights into regional preferences or demographic trends. This spatial perspective adds depth to data analysis, enabling organizations to uncover hidden opportunities or risks that may be region-specific.
Analyzing Location-Based Information
Analyzing location-based information entails examining data within the context of specific geographical regions or coordinates. By overlaying datasets onto maps or geographic grids, analysts can discern spatial relationships and dependencies that inform strategic decision-making. Understanding the influence of location on data patterns provides organizations with a more nuanced perspective on market dynamics, resource allocation, and customer behavior, facilitating targeted interventions and tailored strategies.
Visualizing Spatial Relationships
Visualizing spatial relationships through interactive maps and visualizations fosters a deeper understanding of spatial connections and dependencies. By illustrating how different data points relate to one another in a geographical context, organizations can derive valuable insights into spatial proximity, clustering, or dispersion. This visualization technique enables stakeholders to explore spatial patterns intuitively and make data-informed decisions that consider the spatial dimension of the information at hand.
Tools and Platforms for Data Visualization
In the realm of big data visualization, the utilization of tools and platforms holds paramount importance. These tools serve as the foundation for transforming complex data into comprehendible insights. By employing sophisticated software, analysts can streamline the process of interpreting massive datasets, enabling the extraction of valuable information efficiently. Moreover, these platforms often come equipped with features that cater to various visualization techniques, enhancing the overall data representation experience. When considering tools and platforms for data visualization, factors such as user-friendly interface, scalability, and compatibility with different data sources play a significant role in determining their effectiveness.
Popular Data Visualization Tools
Tableau
Tableau stands out as a frontrunner in the realm of data visualization tools due to its robust features and intuitive interface. The key aspect of Tableau lies in its ability to generate interactive and visually appealing dashboards and reports, facilitating data analysis and interpretation. Its unique feature of drag-and-drop functionality simplifies the process of creating complex visualizations, making it a popular choice among data analysts and business professionals. However, while Tableau offers extensive customization options, its licensing costs and steep learning curve might pose challenges for novice users.
Power BI
Power BI, Microsoft's business intelligence tool, plays a crucial role in data visualization by providing seamless integration with various data sources. Its standout characteristic lies in its advanced data modeling capabilities, enabling users to create insightful visual representations effortlessly. With features such as natural language querying and real-time dashboards, Power BI simplifies the generation of interactive reports, making it a sought-after tool for organizations seeking dynamic data visualization solutions. Despite its strengths, Power BI may have limitations in handling highly complex datasets and intricate data transformations.
QlikView
Qlik View distinguishes itself through its associative model, allowing users to explore data relationships dynamically. The key characteristic of QlikView is its in-memory data processing engine, which accelerates data analysis and visualization tasks. This feature-rich tool empowers users to develop interactive and personalized dashboards, fostering a data-driven decision-making culture within organizations. However, the initial setup complexity and resource-intensive nature of QlikView may pose challenges for inexperienced users.
D3.js
D3.js, a Java Script library, revolutionizes data visualization by offering unparalleled flexibility and customization options. The unique feature of D3.js lies in its support for creating bespoke data visualizations using scalable vector graphics (SVG). Its advantages include the ability to manipulate data-driven documents dynamically and integrate intricate data sets seamlessly. However, the learning curve associated with D3.js can be steep, requiring a solid understanding of web technologies and programming languages for optimal utilization.
Integration with Business Intelligence
Enhancing Analytics Capabilities
Enhancing analytics capabilities through seamless integration with business intelligence tools is pivotal for unlocking actionable insights from big data. By leveraging advanced analytical features, organizations can gain deeper visibility into data trends and patterns, empowering data analysts to derive meaningful conclusions. The key characteristic of this integration lies in its ability to consolidate data from disparate sources and generate comprehensive analytical reports, enabling data-driven decision-making with precision. However, challenges such as data compatibility issues and integration complexities may arise, necessitating thorough planning and optimization to harness the full potential of this synergy.
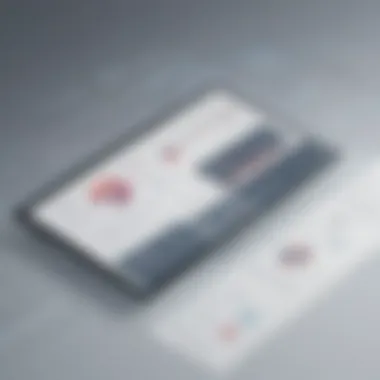
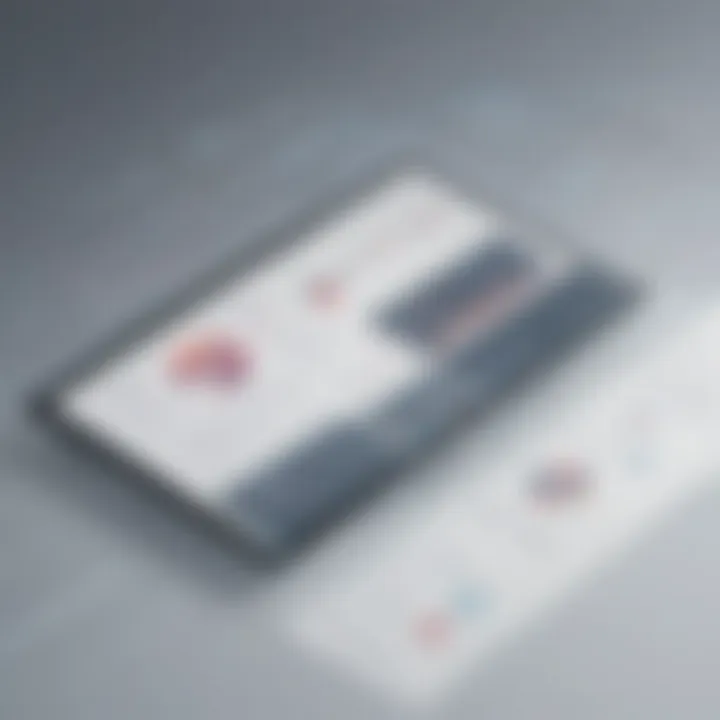
Enabling Data-Driven Decision-Making
Enabling data-driven decision-making through integrated data visualization solutions enhances operational efficiency and strategic planning. The crucial aspect of this process is to empower stakeholders with visual insights that drive informed decision-making based on real-time data analysis. By facilitating interactive data exploration and fostering collaboration across departments, organizations can prioritize data-driven strategies and actions. The unique feature of this integration is its ability to cultivate a data-driven culture, where decisions are backed by evidence and analysis, promoting business growth and innovation. However, ensuring data accuracy and aligning with organizational objectives are critical considerations for successful implementation.
Creating Interactive Reports
Creating interactive reports within the business intelligence framework is instrumental in presenting data in a cohesive and engaging manner. The key characteristic of interactive reports is their dynamic nature, allowing users to interact with data elements and explore insights intuitively. By incorporating features such as drill-down functionalities and customizable dashboards, interactive reports facilitate in-depth data analysis and visualization, empowering users to uncover hidden trends and correlations efficiently. The unique feature of interactive reports is their capacity to deliver real-time insights and foster data-driven conversations among stakeholders. However, balancing user interface complexity with data comprehensibility remains a challenge, requiring iterative design enhancements and user feedback for optimal report creation.
Challenges and Considerations in Big Data Visualization
In the expansive landscape of big data visualization, navigating the challenges and considerations inherent in this process becomes paramount. Understanding the complexities of handling large data sets is vital for extracting meaningful insights. Scalability and performance issues emerge as significant factors influencing the efficacy of visualization techniques. The ability to scale resources and maintain optimal performance when dealing with vast amounts of data distinguishes successful visualizations. Scalability ensures adaptability to varying data volumes, while performance addresses the speed and efficiency of data processing. However, challenges such as resource limitations and processing bottlenecks can hinder scalability and performance, impacting the overall visualization quality.
Data aggregation and sampling play a pivotal role in streamlining data processing and analysis. By aggregating data, organizations can condense vast datasets into manageable units, simplifying visualization tasks. Conversely, sampling involves selecting representative subsets of data to derive insights without processing the entire dataset. While data aggregation accelerates processing speed and reduces complexity, sampling allows for quick analyses but may introduce sampling errors. Balancing between aggregation and sampling methodologies is crucial to ensure data accuracy and efficiency in visualization practices.
Maintaining Data Accuracy and Integrity
The integrity of data is essential in driving informed decision-making and ensuring the reliability of insights derived from visualizations. Data quality assurance procedures are implemented to validate the accuracy, completeness, and consistency of datasets. By adhering to quality assurance standards, organizations can mitigate the risks associated with erroneous data interpretations. Data quality assurance involves thorough data validation checks, error detection mechanisms, and data cleansing processes to uphold data integrity throughout the visualization pipeline.
Preventing misinterpretation of data is a fundamental objective in data visualization projects. Misinterpretations can lead to inaccurate insights, impacting strategic decisions and organizational outcomes. Implementing measures to prevent misinterpretation involves employing clear data labeling, contextualizing data points, and providing comprehensive data documentation. By enhancing data clarity and context, organizations can reduce the likelihood of misinterpretations and ensure stakeholders interpret visualizations accurately.
User Interface Design and User Experience
The design of intuitive visualization interfaces contributes significantly to user engagement and comprehension of visualized data. Intuitive interfaces prioritize user-friendly interactions, seamless navigation, and clear data presentation. By simplifying complex data structures into visually appealing formats, intuitive interfaces enhance user experience and promote data exploration. Incorporating interactive elements and customizable features allows users to interact with data dynamically, fostering a more immersive and personalized visualization experience.
Optimizing user interaction involves tailoring visualization platforms to accommodate varying user preferences and requirements. Customizing user interactions enhances user satisfaction and efficiency in interpreting visualized data. Features such as interactive filters, customizable dashboards, and personalized data views empower users to manipulate data presentations according to their needs. By optimizing user interactions, organizations can improve data accessibility, foster user engagement, and facilitate data-driven decision-making processes.
Emerging Trends in Big Data Visualization
Emerging Trends in Big Data Visualization are pivotal to this article, offering insights into cutting-edge advancements reshaping the realm of data representation. The incorporation of innovative technologies like Augmented Reality and Virtual Reality is revolutionizing data visualization practices. By leveraging Augmented Reality and Virtual Reality, organizations can delve deeper into their datasets, unveiling hidden patterns and gaining unique perspectives. These emerging trends bring a new dimension to data visualization, enhancing decision-making processes and fostering comprehensive data analysis. Embracing these trends equips professionals with powerful tools to extract valuable insights from complex data landscapes.
Augmented Reality and Virtual Reality
Immersive Data Visualization Experiences
Discussing Immersive Data Visualization Experiences within the context of this article reveals a distinctive approach to data interpretation. Immersive Data Visualization facilitates a sensory-rich environment where users can interact with data in a virtual setting, promoting enhanced engagement and understanding. The immersive nature of this experience engrosses users in data exploration, leading to deeper insights and informed decision-making. Its ability to simulate real-world scenarios elevates the data visualization process, making it an invaluable asset for professionals navigating vast datasets. Despite its immersive advantages, challenges like hardware requirements and sensory overload may hinder its adoption but overcoming these obstacles leads to unparalleled visual data comprehension.
Enhancing Data Exploration
Exploring how Enhancing Data Exploration contributes to data visualization underscores its significance in uncovering comprehensive insights. Enhancing Data Exploration empowers users to navigate through data sets seamlessly, enabling them to uncover intricate relationships and patterns efficiently. The key characteristic of this aspect lies in its user-friendly interface that simplifies complex data structures, making exploration intuitive and informative. By providing users with tools to interact dynamically with data, Enhancing Data Exploration streamlines the visualization process, enhancing efficiency and understanding. While Enhancing Data Exploration offers unparalleled data exploration capabilities, ensuring data security and privacy during exploration remains a critical consideration in this article, emphasizing the need for robust protection measures.
Machine Learning for Enhanced Visualization
Automating Pattern Recognition
Delving into Automating Pattern Recognition illuminates its pivotal role in streamlining data analysis and visualization. By automating pattern recognition tasks, Machine Learning algorithms identify patterns within datasets swiftly, enhancing data processing speed and accuracy. The key characteristic of Automating Pattern Recognition lies in its ability to extract meaningful insights from vast data volumes efficiently, enabling professionals to focus on strategic decision-making. This automated process accelerates pattern identification, empowering organizations to uncover hidden trends and correlations effortlessly. While Automating Pattern Recognition optimizes data analysis, ensuring algorithm transparency and interpretability are crucial factors to consider, safeguarding against bias and misinterpretation in data-driven decisions.
Predictive Data Analysis
Examining the realm of Predictive Data Analysis within the context of this article sheds light on its transformative impact on data visualization. Predictive Data Analysis utilizes Machine Learning algorithms to forecast future trends based on historical data patterns, aiding in predictive modeling and decision-making. The key characteristic of Predictive Data Analysis lies in its predictive accuracy and scalability, offering organizations invaluable insights into future outcomes. Leveraging Predictive Data Analysis enhances forecasting capabilities, enabling proactive decision-making and risk management. While Predictive Data Analysis provides unprecedented predictive insights, continuous model evaluation and refinement are necessary to ensure the reliability and relevance of predictive outcomes in this article.